Geoscience Reference
In-Depth Information
(a)
(b)
9
9
8
8
7
7
6
6
5
5
4
4
3
3
2
2
1
1
0
0
Figure 3.11
Median DOC concentration (mg l
−1
) of surface waters in the UK Acid
Water Monitoring Network, 1988-2007. (a) Lakes. (b) Streams. Bars show the 25th and
75th percentile concentrations at each sampling interval.
0.8
0.6
0.4
0.2
0.0
Q+
SO
4
SO
4
Temp
CI
Q
All var
-0.2
Figure 3.12
The variance in OM explained by the different potential drivers. Box-plot
(10th, 25th, 50th, 75th, and 90th percentiles marked) of the
r
2
-values for COD, modelled
with linear regression for 28 rivers, using temperature, [Cl
−
], [SO
4
2−
] and flow as
explanatory variables. Combinations of these parameters were modelled using multiple
linear regression. (Modified from Erlandsson
et al
. 2008.)
by increasing the proportion of DOC-rich water derived from the upper organic
horizons of mineral soils and secondly by reducing water residence time, and
hence DOC removal, in lakes (Hongve
et al
. 2004). Rising levels of atmospheric
CO
2
, influencing plant growth and litter quality were also proposed to explain
increased rates of DOC production (Freeman
et al
. 2004).
Erlandsson
et al
. (2008) showed that for the 35-year period (1970-2004), most
of the inter-annual variability of concentrations of dissolved organic matter (OM)
in 28 large Scandinavian river catchments were explained by discharge and
concentrations of sulphate, with discharge being the more important driver.
Despite the heterogeneity of the catchments with regards to climate, size and land
use, there was a high degree of synchroneity in chemical oxygen demand (COD),
a common proxy for OM, across the entire region. Multiple regression models
with discharge and sulphate concentration explained up to 78% of the annual
variability in COD, while two other candidate drivers, air temperature and
chloride concentration in river water, added little explanatory value (Fig. 3.12).













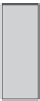



















































































Search WWH ::

Custom Search