Graphics Reference
In-Depth Information
We use these two vertices and the selected boundary vertices construct the control
cage of the source part.
Size Aware Deformation.
Assume that the source part is S and the target part is T,
we automatically fit the source S to the target T by computing the ratio k of source's
boundary length and target's boundary length. Then we construct a new cage using
the selected vertex in the target mesh, and add two vertices with length of kR in the
same way as source cage dose. We deform the source part using the new control
cage and interpret with the mean value coordinate computed by the old cage. The
source mesh is stitched to the target mesh finally. See Fig.3.
6
Result and Discussion
We train the probabilistic graphic model on a cloth image dataset crawled from online
shop. The cloth image contains five garment style, T-shirt, shirts, skirt, coat and suit.
The learned structure is showed in Fig.2. The learned conditional probability of
component node is showed in Table 1. Fig.4 shows that the learned Bayesian graphic
model increase the relevance of presented components than static ordering.
Some of the generated garment model is showed in Fig.5. As we established a one
to one mapping of the boundary vertex, garment components with border like skirt,
collar and sleeve are merged into garment body seamlessly. Components are
adaptively scaled to fit the garment body without distortion. Our cage-deformation
method adds a control vertex in the normal direction; it can preserve well the surface
detail feature of garment components well. Our deformation method adopts mean
value coordinate to interpolate the new position of vertex, so it can also be applied to
assemble components with complex topology like button and belt.
Fig. 4.
The figure shows the recall of components, the probabilistic model presented more
relevant components than the static ordering
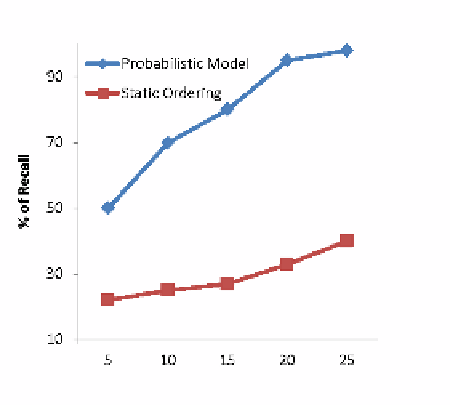
Search WWH ::

Custom Search