Information Technology Reference
In-Depth Information
a
b
c
30
80
40
60
30
20
40
20
10
20
10
0
0
0
−10
−5
0
5
10
−10
−5
0
5
10
−10
−5
0
5
10
Fig. 19.4
Level of conflict (in the range
Œ10; C10
) histograms for the Challenge partitions of
the SSPNet Conflict Corpus. (
a
)train,(
b
)devel,(
c
)test
Table 19.2
Low-level
descriptors (LLD) of features
set I
Energy-related LLD
Sum of auditory spectrum (loudness)
Sum of RASTA-style filtered auditory spectrum
RMS energy
Zero-crossing rate
Spectral LLD
RASTA-style auditory spectrum, bands 1-26 (0-8 kHz)
MFCC 1-14
Spectral energy 250-650 Hz, 1k to 4kHz
Spectral roll off point 0.25, 0.50, 0.75, 0.90
Spectral flux, entropy, variance, skewness, kurtosis,
Slope, psychoacoustic sharpness, harmonicity
Voicing-related LLD
F0 by SHS + Viterbi smoothing, probability of voicing
logarithmic HNR, spectral harmonicity
Psychoacoustic spectral sharpness
Jitter (local, delta), shimmer (local)
19.5
Feature Sets
In this paper we investigate the use of three fundamentally different sets of features:
Feature set I
,alsoreferredtoas
baseline feature set
, is a supra-segmental feature
set, in our case one segment per utterance. This is an approach often followed
in emotion recognition and paralinguistic analysis. For this purpose we adopt the
baseline acoustic feature set used in the ComParE Conflict Sub-Challenge (Schuller
et al.
2013
). We use the open-source feature extractor openSMILE (Eyben et al.
2010
), developed by the Technical University of Munich, to extract the features
on a per-chunk level. The extracted features are the ones already proposed in
the Interspeech 2012 Speaker Trait Challenge (Schuller et al.
2012
) with some
additional modifications and features. First, so-called low-level descriptors (LLD)
are extracted, which are listed in Table
19.2
.


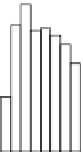




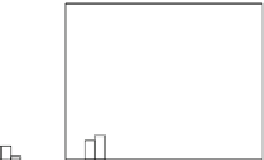

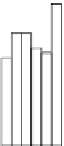











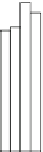


























































































Search WWH ::

Custom Search