Geoscience Reference
In-Depth Information
72 CHAPTER 7. HUMAN SEMI-SUPERVISEDLEARNING
where low
new
is the low-variance distribution around
μ
new
.
Therefore, because of the new mode at
μ
new
in
test data
, human subjects perceive
μ
new
to be
more likely in class 1. This experiment clearly demonstrates that humans do not fix their hypothesis
after training—unlabeled test data influences humans' learned hypotheses. It is worthy pointing
out that the observed behavior cannot be simply explained by a change in the threshold
in (7.1).
Instead, it likely involves the change in the conditional density
p(
x
|
y
=
1
)
.
40
μ
(a) a stimulus
(b) training distribution
4
4
1
20
20
2
20
19
40
40
μ
μ
new
μ
(c) condition 1 test distribution
(d) condition 2 test distribution
Figure 7.1:
The one-class classification problem of Zaki and Nosofsky.
7.3
STUDYTWO: PRESENCEOFHUMANSEMI-SUPERVISED
LEARNING INA SIMPLETASK
Zhu, Rogers, Qian and Kalish (ZRQK) conducted an experiment that demonstrates semi-supervised
learning behavior in humans [
214
]. Unlike the Zaki and Nosofsky study, it is a binary classification
task. We call it a “simple task” because the feature space is one-dimensional, and there is a single
decision boundary (threshold) that separates the two classes. In the next section, we will discuss a
more complex task in two dimensions.
Their experiment design is shown in Figure 7.2(a), which is similar to Figure 2.1 earlier in
the topic. There is a negative labeled instance at
x
=−
1 and a positive labeled instance at
x
=
1.
=
From these labeled instances alone, supervised learning would put the decision boundary at
x
0.
Suppose in addition, the learner observes a large number of unlabeled instances, sampled from
the blue bi-modal distribution. Then many semi-supervised learning models will predict that the
decision boundary should be in the trough near
x
=−
0
.
5.Therefore, supervised and semi-supervised
learning models lead to different decision boundaries.








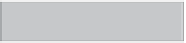

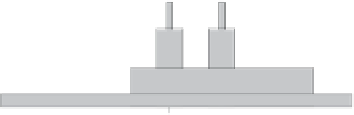





