Geoscience Reference
In-Depth Information
justice to some of the many faces of uncertainty. For simplicity, the high level
classification by Klir and Folger (1988) of
ambiguity
(one-to-many relationships) and
vagueness
(or fuzziness) is preferable, but readers are advised to refer to the above
citation if a more elaborate classification is intended. Their high level classification into
ambiguity and vagueness coincides with that of Ayyub and Chao (1998). Ayyub (2001)
defined
ambiguity
as the possibility of having multi-outcomes for processes or systems,
and
vagueness
as noncrispness of belonging and nonbelonging of elements to a set or a
notion of interest. The ambiguity type of uncertainty also includes the most appreciated
random
uncertainty (see Johnson and Ayyub, 1996). Owing to the dominant application
of probability and fuzzy set theories in modelling uncertainty, uncertainty is also
classified as
random
and
fuzzy
(e.g. Kaufmann and Gupta, 1991; Reddy and Haldar,
1992). In this thesis, in referring to uncertainty represented by probability and fuzzy set
theories the random and fuzzy types of uncertainty are frequently used.
In the context of modelling engineering systems, the classification of uncertainty into
inherent
and
epistemic
(see, e.g. Van Gelder, 2000) is also very useful and appealing. The
inherent
uncertainty is the variability or randomness in space and time and the
epistemic
uncertainty is due to the lack of knowledge of fundamental phenomena. The latter type is
further divided into statistical (parameter and distribution type) and model uncertainty.
Uncertainty is also sometimes referred to as
quantitative
and
qualitative
. The former type
refers to the uncertainty when it is represented using numerical values, typically by
statistical inferences. Uncertainty if it is represented using linguistic variables is referred
to as qualitative uncertainty.
2.2.2
Sources of uncertainty in flood forecasting
The sources of uncertainty refer to the causes that give rise in uncertainty in the
forecasted state of a system that is modelled. In the context of flood forecasting, the
sources of uncertainty are classified as (Maskey et al., 2003b):
1. Model uncertainty (due to assumptions in model equations, model building, and
other forms of incompleteness in conceptualising the real system)
2. Input uncertainty (due to imprecise forecasts of and/or uncertainty in model
inputs, such as future precipitation, evaporation, etc.)
3. Parameter uncertainty (due to imperfect assessment of model parameters)
4. Natural and operational uncertainty (due to unforeseen causes, e.g. glacier
lake overflow, dam break, landslides, etc., malfunctioning of system components
(hardware and software), erroneous and missing data, human errors and mistakes, etc.).
The above classification for the sources of uncertainty retains some features of the
classification by Van Gelder (2000). Some other classifications that closely correspond to
this classification are Kitanidis and Bras (1980): model uncertainty, input uncertainty,
parameter uncertainty and initial state of the system; Melching (1995): natural
randomness, data uncertainty, model parameters and model structure; and Krzysztofowicz
(1999): input uncertainty, hydrological uncertainty and operational uncertainty.
The fourth category of the sources of uncertainty listed above is broad and is usually
not addressed by uncertainty assessment in operational flood forecasting systems. The
parameter uncertainty can reflect part of the uncertainty associated with the model
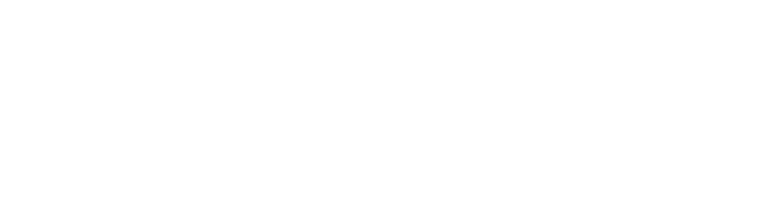
Search WWH ::

Custom Search