Information Technology Reference
In-Depth Information
Legend:
Fig. 1.
Raw data streams and preprocessing procedures forming secondary data sources consti-
tuting input data streams of IDS
(1)
Connection-related
data that are used for extraction of connection-related fea-
tures forming two data sources, i.e.
ConnectionBased
and
ContentBased
data sources.
(2)
Time window-related
data representing certain statistics averaged within sliding
time window of the predetermined length and shift (in our case,
length
=
5 sec
. and
shift=2 sec
.). These data are used for extraction of the features forming two secondary
data sources,
TimeWindowFeatures
, and
TimeWindowTrafficFeatures
.
(3)
Connection window-related
data representing certain statistics averaged within
sliding time window containing a user-assigned number of connections (in our case,
this number is equal to 20 connections and shift is equal to 1 connection). These data
are used for extraction of the features forming two more secondary data sources,
ConnectionWindowFeatures
, and
ConnectionWindowTrafficFeatures
.
Traffic preprocessing procedures were developed by authors. As the input of these
procedures, the DARPA data [3] are used.
2.2 Heterogeneous Alert Correlation Structure
The primary factor influencing on the IDS architecture is the structure of interaction
of the source-based classifiers and meta-classifiers. Let us comment it by example of
the structure used in the developed case study illustrated in Fig. 2.
Each data source is attached several source-based classifiers. A peculiarity of
these classifiers is that each of them is trained for detection of a fixed class of attacks
and produces alerts regarding corresponding attack class. That is why the alerts pro-
duced are heterogeneous, i.e. correspond to different classes of attacks. Actually,
each source-based classifier solves an anomaly detection task, but each "anomaly"
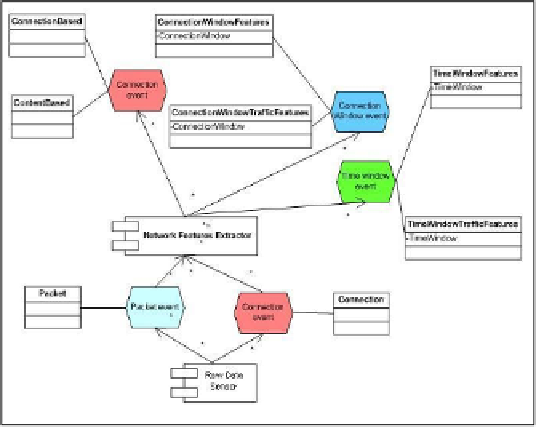
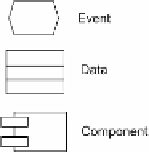