Geology Reference
In-Depth Information
The GPD tail model can be used to make predictions regarding rare events which
are farther out in the tail than any of the data we used to compute the GPD model.
This can be done by the important distribution of the EVT, which refers to GPD. It
can be de
ned as
8
<
1
=
k
kz
a
1
1
þ
;
k
6
¼
0
G
a
;
k
ð
z
Þ
¼
ð
8
:
2
Þ
:
e
z
=
a
1
;
k
¼
0
Finally, we can fit GPD to the conditional CDF [
3
,
12
].
In the above basic
flow, the classi
er plays an important role. One of the popular
classi
ers are Support Vector Machines (SVMs) [
6
]. These were created by
Vladimir Vapnik in 1992 and are a powerful machine learning technique which can
be used for both regression and classi
cation problems (in our SB method). This
method uses theory and techniques from machine learning and statistical theory
which were already well known. Support vector regression (SVR) is a special type
of SVM. The success of classi
ers and prediction methods depends heavily on the
quality and choice of the data used. Domain knowledge is important to be able to
choose features likely to bring good results. The standard SVM is used for clas-
si
cation and will be introduced brie
y.
For clarity, here we consider two input variable classi
cation. In Fig.
8.2
, where
there are two input features x =(x1, x2) and three possible classes denoted by
symbols such as o, * and []. A classi
er is a function C(x) which, given some input
1
0.8
0.6
0.4
0.2
0
-0.2
-0.4
-0.6
-0.8
-1
-1
-0.8
-0.6
-0.4
-0.2
0
0.2
0.4
0.6
0.8
1
x1
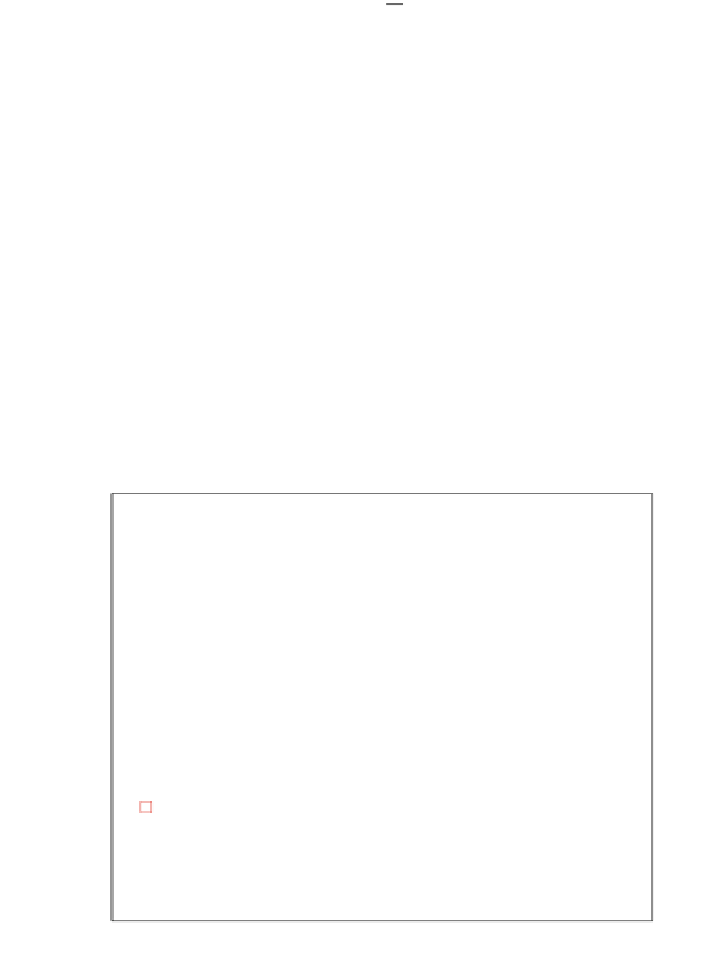






























































































































































































































































































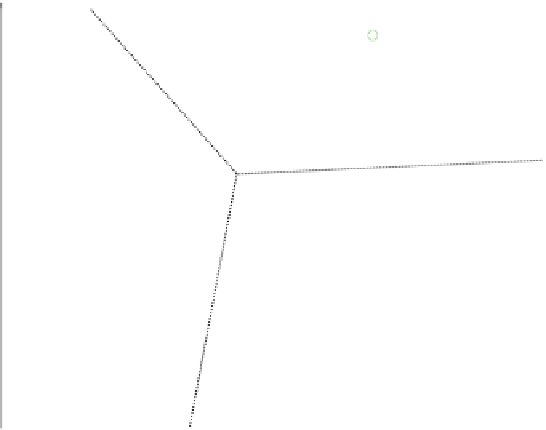





















































































































































































































































































































































