Geology Reference
In-Depth Information
Fig. 5.33 Variation of RMSE in
ʵ
-SVM with linear kernel with cost factor of error (C) in daily
solar radiation modelling
10 since it produced the least root means square error, while being the most eco-
nomical on computing processing.
The SVM hypothesis suggests that the performance of SVM depends on the
slack parameter (
ʵ
). To
find the optimum slack parameter, we have performed the
analysis varying the
ʵ
values between
ʵ
=1to
ʵ
= 0.00001. The analysis results on
variation of slack parameter (
) are shown in Fig.
5.34
. The Figure has shown that
the least error is the lowest when the
ʵ
ʵ
value is 1. So we set the value of
ʵ
to 1 for
reliable results and less computation time.
The modelling results of SVM model (
-SVR with linear kernel function) during
training phase is shown in Fig.
5.35
a, b. The validation results of SVM model is
given in Fig.
5.36
a, b.
The statistical performance of ANFIS model with the support vector machine
(SVM) technique with epsilon SVR and linear kernel is presented in Table
5.2
.
ʵ
Fig. 5.34 Variation of RMSE in
ʵ
-SVM with linear kernel with epsilon value (
ʵ
) in daily solar
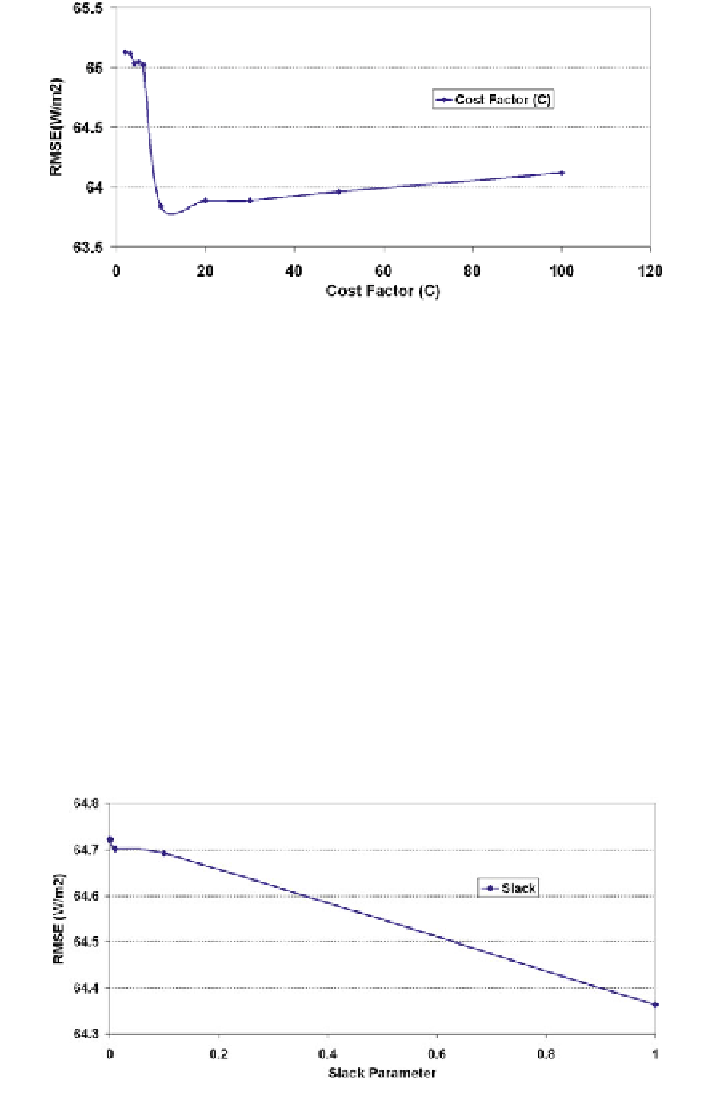