Biology Reference
In-Depth Information
Numerous other more complex distance measures are being used in
research studies, and no single standard has emerged yet. As different
dissimilarity measures will often change the clustering of the microarray
data, comparison of results across different measures should not be
made.
E
XERCISE
12-2
Show that d
E
ð
x
i
;
x
k
Þ
from Eq. (12-3) satisfies the distance axioms 1
through 3.
Hint: First prove the result for n
¼
2.
E
XERCISE
12-3
Show that d
B
ð
x
i
;
x
k
Þ
from Eq. (12-5) satisfies the distance axioms 1
through 3.
E
XERCISE
12-4
Show that d
C
ð
x
i
;
x
k
Þ
from Eq. (12-6) satisfies the distance axioms 1
through 3.
C. Cluster Analysis Methods
Gene ''Name''
Tissue Types
1
2
1. Hierarchical clustering
Consider the set of gene expression values shown in Table 12-3. For this
illustration, we consider the gene expression values measured for only
two hypothetical tumor samples. In this case, n
A
1.5
0.4
B
1.4
0.5
2, and the expression
values can be depicted as points on a two-dimensional coordinate
system. We can also label the rows of the data matrix X by the gene
names A, B, C,
¼
C
1.1
0.3
D
1.2
0.5
E
1.4
0.8
. Now, the Euclidean distance
from Eq. (3) is the usual geometric distance between points in the plane.
For our example,
, instead of x
1
,x
2
,x
3
,
...
...
F
1.6
0.2
TABLE 12-3.
Gene expression values for a set of hypothetical
tumor sampling.
q
ð
p
0
2
2
d
ð
A
;
C
Þ¼
1
:
5
1
:
1
Þ
þð
0
:
4
ð
0
:
3
ÞÞ
¼
:
17
¼
0
:
412
:
See Figure 12-10.
After the distances between any two rows of the data matrix X are
computed, it is convenient to store the data again as a matrix, called
a proximity matrix. The proximity matrix for the data in Table 12-3 is:
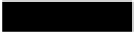

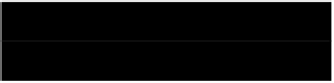

























