Environmental Engineering Reference
In-Depth Information
(a)
10
4
y
= 1.3888
x
0943
R
2
= 0.978
Samples
100.00
10
3
10.00
1.00
10
2
0.10
Measured SSC (mg/L)
Predicted SSC (mg/L)
10
1
0.01
0
20 40
Frequency of exceedence (%)
60
80
100
10
0
10
0
10
2
Turbidity from fixed-location, in-stream monitor,
in formazin nephelometric units
(YSI 6026 turbidity sensor)
10
1
10
3
10
4
(b)
10000
Sample
Fig. 1.8
Comparison of fi eld turbidity in formazin
nephelometric turbidity units and SSC for the Little Arkansas
River at Sedgwick, Kansas, USA, 1999-2006.
1000
100
10
retransformed to their original units, a step that
introduces a bias (usually negative) in computed SSC
values (Miller 1951; Koch & Smillie 1986) unless the
data are perfectly and positively correlated. To
correct for retransformation bias, Duan (1983)
introduced a nonparametric bias-correction factor
called the “smearing” estimator. Duan's (1983)
smearing estimator is insensitive to non-normality in
the distribution of regression residuals about a loga-
rithmically transformed model. A method proposed
by Cohn
et al
. (1989) assumes normally distributed
residuals about the logarithmic model and results in
an exact minimum variance unbiased estimator and
its variance.
Schoellhamer
et al
. (2002) describe a successful
multi-station, multi-year fi eld investigation in
California's San Francisco Bay and Delta system.
OBS sensors at each station are calibrated with SSC
from water samples collected at each site. San
Francisco Bay OBS sensors are calibrated to point
samples (described in Section 1.1) and San Francisco
Delta OBS sensors are calibrated to discharge-
weighted, cross-sectionally averaged SSC values. SSL
is determined by multiplying the discharge-weighted,
cross-sectionally averaged SSC by water discharge,
accounting for tide-driven bi-directional fl ow
(Schoellhamer
et al
. 2002).
1
0
20 40
Frequency of exceedence (%)
60
80
100
Fig. 1.7
Duration curves for (a) streamfl ow, and (b) turbidity
measured in samples collected in the Little Arkansas River at
Sedgwick, Kansas, USA, 1999-2005.
observed turbidity, SSC, and streamfl ow values for
the site is paramount in developing a reliable model.
Simple linear regression analysis explained in
Rasmussen
et al.
(2009) was used to develop a site-
specifi c univariate model using turbidity to compute
time-series SSC (Fig. 1.8). The model explains about
98% of the variance in SSC. Continuous SSLs com-
puted from the model and paired water discharge-
SSC time-series datasets are available online (USGS
2005).
Base-10 logarithmic transformation is one of
several mathematical functions that can be used to
transform datasets to meet the assumptions for linear
regression analysis. Other considerations should
include the ease of retransforming the results from
the model and the bias associated with the retrans-
formation. The computed SSC values must be

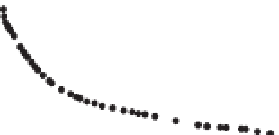




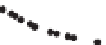

























