Information Technology Reference
In-Depth Information
pairwise comparisons for all levels of the decision tree and, within each level, to
obtain weights for criteria. This makes its application time consuming. In addition,
AHP does not deal with the problem of dependencies between more than two
concerns because of its pairwise structure.
Hence, we decided to compare the AHP method with a widely known direct
scoring method such as the classical weighted average (1), using the same illustrative
example. Obviously, with this method we lose the possibility of using pairwise
judgment and checking the logical consistency of the judgements made, but we gain
in terms of time spent. In addition, with the simple WA we can neither compare the
intermediate values nor use direct pairwise judgements to express our preferences
between each pair of concerns, since in the classical weighted average method the
assigned importance are direct (it is a direct scoring method).
In spite of the drawbacks of using a simple weighted average approach for our type of
problem, it is a widely used method and a good candidate for comparison purposes.
Furthermore, we will use a classification scale within the interval [0, 1] to enable a direct
comparison with the final results of the AHP method. To allow some interpretation of the
classification of alternatives we will use a discrete set of values from the interval scale,
such as: {Very important: 0.9, Important: 0.75, Medium: 0.5, Low: 0.3, Very low: 0.1}.
This classification is based on discrete fuzzy sets, as described in [10]. Table 16 shows
the weights (i.e., the relative importance of criteria).
Table 16.
Criteria weights
Table 17 shows the classifications for each alternative per criterion, the final
priority obtained with Eq. (1), and the respective final ranking.
Table 17.
Alternatives classification, final priorities and ranking for Enter Subway match point






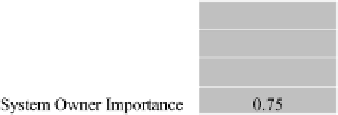






























































Search WWH ::

Custom Search