Information Technology Reference
In-Depth Information
m
1
=
m
−
1
=
2
w
T
(
∂V
∂w
0
2
σ
(
m
−
1
+
m
1
)=0
−
μ
1
−
μ
−
1
)
=
⇒
.
(3.59)
1
2
w
T
(
w
0
=
−
μ
1
+
μ
−
1
)
The critical points of
V
do indeed constrain the discriminant to pass through
the point lying half-way of the means. Let us now rotate the coordinate
system of
X
such that one of the coordinates, say
k
, passes through the mean
vectors
1
μ
−
1
)=0; therefore, setting
w
0
=0.
This rotation and shift amount to an additional linear transformation of the
inputs. Since in the new coordinate system,
μ
t,i
=0and
μ
1
,k
=
μ
t
, and shift its origin to
2
(
μ
1
+
−
μ
−
1
,k
=
μ
,
we then have:
2
σ
(1 +
w
k
μ
2
)+
σ.
V
=
(3.60)
For the partial derivatives relative to the weights
w
i
, we distinguish two cases:
1.
i
=
k
:
∂V
∂w
i
w
i
σ
3
(
σ
2
2
w
k
μ
2
)=0
.
=0
⇒
−
2
−
(3.61)
For
σ
=0,thesolutionsare
w
i
=0
,i
=
k
,and
σ
2
=2+2
w
k
μ
2
.
2.
i
=
k
:
∂V
∂w
k
w
k
σ
3
(
σ
2
(1 + 4
μ
2
)
2
w
k
μ
2
)=0
.
=0
⇒
−
2
−
(3.62)
=0,thesolutionsare
w
k
=0and
σ
2
(1 + 4
μ
2
)=2+2
w
k
μ
2
.
Combining the solutions of 1. and 2. the critical points, for
σ
=0and
μ
=0,
are readily seen to be:
For
σ
2
1+2
μ
2
;
w
i
=
k
=0
,w
k
=
±
(3.63)
w
k
=0
,
i
=
k
w
i
=2
.
(3.64)
The solution
w
i
=
k
=0(3.63) corresponds to a hiperplane orthogonal to the
vector linking the means, i.e., to the Bayes solution for whitened inputs. Once
this hyperplane has been found, we may revert to the original system of coordi-
nates and apply the inverse whitening transformation (
Λ
1
/
2
Φ
T
)
T
x
.Wethen
get expression (3.57) because
x
T
x
transforms into
s
T
ΦΛ
−
1
/
2
Λ
−
1
/
2
Φ
T
s
=
s
T
Σ
−
1
s
since
Φ
T
=
Φ
−
1
and
Σ
−
1
=(
ΦΛΦ
T
)
−
1
=(
Φ
T
)
T
Λ
−
1
Φ
T
.
The type of the critical points (3.63) and (3.64) is determined by the eigen-
values of the Hessian. First note that in (3.60),
V
does not depend on the
bias. For the weights, setting
A
=1+
w
k
μ
2
and
B
=1+4
μ
2
:














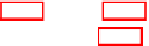