Information Technology Reference
In-Depth Information
IG
TWO
1
1
β
β
0.5
0.5
0
0
α
α
0
0.5
1
0
0.5
1
PE
EE
1
1
β
β
0.5
0.5
0
0
α
0
0.5
1
0
0.5
α
1
Fig. 4.13
The
p
=0
.
5
setting. Darker tones and larger sizes correspond to higher
values.
prefers purer nodes. Cases 'b' and 'd' constitute one further illustration of
the sensitivity of
SEE
to
PE
,nowinthe
α
=
β
context. Similar conclusions
can be drawn for other
p
∈
]0
,
1[ configurations.
Tabl e 4 . 3
Criteria values for Fig. 4.14 configurations.
αβIGTWOPESEE
a 0.49 0.01 0.49 0.68 0.75 1.04
b 0.80 0.80 0.50 0.64 0.20 0.64
c 0.60 1.00 0.42 0.57 0.20 0.50
d 0.70 0.89 0.50 0.64 0.21 0.63
To sum up, the empirical MEE (SEE) split behaves according to the the-
oretical properties regarding the class-conditional distribution overlapping,
with the selection of empirical MEE split points generating a high interval-
end hit rate for largely overlapped distributions. The SEE splitting function
is a convenient concave function, as are the classic splitting functions (GI,
IG, and TWO); however, SEE is more sensitive to the error rate, producing
better splits in many simple situations. In Chap. 6 we will analyze how to
use MEE splits in decision trees and what are the possible advantages.
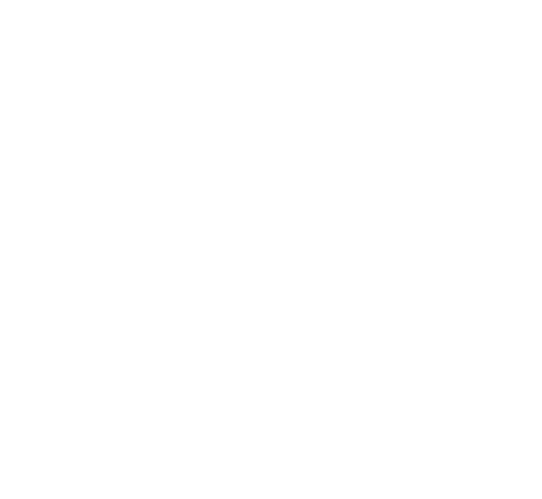
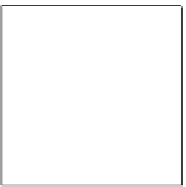













































































































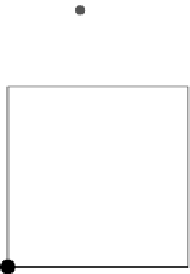














































































































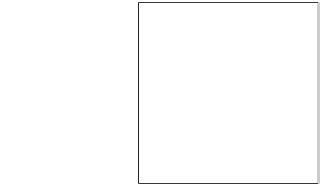

















































































































































































































































































