Biology Reference
In-Depth Information
The success of DNA technologies and the digital revolution brought
about by the growth of the Internet have ensured that huge volumes of
high-dimensional microarray expression data are highly available. Data
mining is an evolving and growing area of research and development. The
problem is to mine useful information or patterns from the huge datasets.
Microarrays provide a powerful basis to monitor the expression of tens of
thousands of genes in order to identify mechanisms that govern the acti-
vation of genes in an organism. Microarray experiments are done to pro-
duce gene expression patterns, which provide dynamic information about
cell function. The huge volume of such data, and their high dimensions,
make gene expression data suitable candidates for the application of data
mining functions.
We have provided an introduction to knowledge discovery from
microarray experimental datasets. The major functions of data mining
have been discussed from the perspectives of machine learning, pattern
recognition, and artificial intelligence.
Soft computing methodologies, involving fuzzy sets, neural networks,
genetic algorithms (GAs), rough sets, wavelets, and their hybridizations,
have recently been used to solve data mining problems. They strive to pro-
vide approximate solutions at low cost, thereby speeding up the process.
For future research and development in microarray data analysis and min-
ing, all of these methods are useful tools. The role of soft computing in
microarray gene expression study is very promising with the learning abil-
ity of neural networks to predict, the searching potential of GAs and SDL,
and the uncertainty-handling capacity of fuzzy sets. On the other hand, by
using an orthogonal array (OA) and other mathematical techniques, it is
practical to develop a global optimization program for cancer classification
and validation on a desktop computer. The primary advantages of this
technique are that the global optimum is always found, excellent solutions
can be found with little prior knowledge, and the new objective functions can
be created according to whatever combination of parameters is required.
The mathematical procedures used in this form of global optimization
are possible to apply to a variety of other previously unsolved problems
relating to the resultant of dependent variables, including experimental
design and manufacturing variations. There are many other approaches
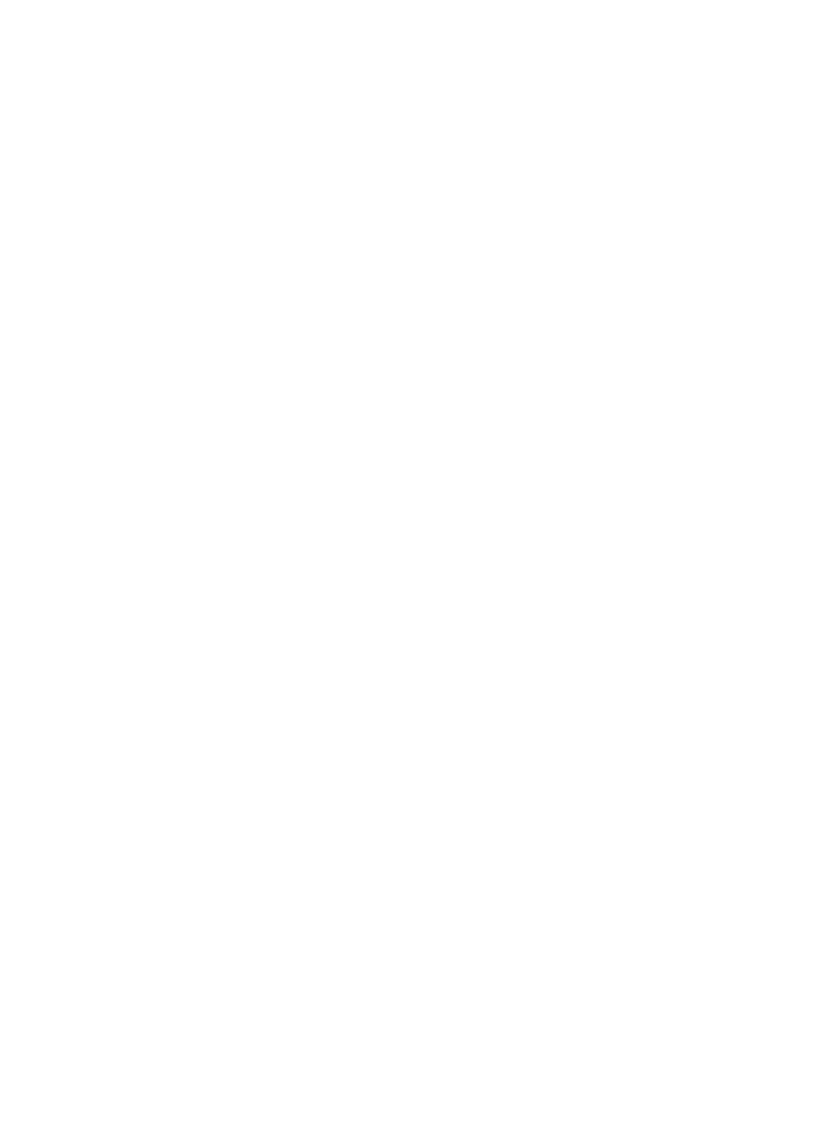
Search WWH ::

Custom Search