Environmental Engineering Reference
In-Depth Information
Fig. 19.10
The standard deviation errors of LST retrieval from the GOES-13 simulations for the
two proposed GOES M (12)-Q algorithms for daytime (
left
) and nighttime (
right
)
To have a closer look at error distributions, we produced errors vs. viewing
angles and skin temperature distributions of the regression fits for the two proposed
GOES M (12)-Q algorithms in Figs.
19.10
,
19.11
,
19.12
, and
19.13
for daytime and
nighttime results, respectively. Figure
19.9
shows the standard deviation error is
usually larger during daytime than those during nighttime. Compared to split-
window-type algorithms as shown in Fig.
19.9
, we can see both dual-window
(3.9 + 11
m) algorithm and one-channel (
T
11
+
T
PW
) algorithms show worse
performance than the split-window-type algorithms (Fig.
19.9
). Meanwhile,
Fig.
19.10
shows that the bias errors from dual-window algorithm are smaller
than those from one-channel algorithm. If we stratify LST retrieval according to
water vapor intervals of 2.0 g/cm
2
, then both standard deviation and bias errors
show improvements than those without water vapor stratification. But one-channel
algorithm still shows worse performance than dual-window algorithm. Both dual-
window and one-channel algorithms still show worse performance than split-
window-type algorithms.
Compared to the daytime algorithm performance, the standard deviation and
bias errors of the nighttime are usually better than that during daytime for each
algorithm. This is because there is no or less solar contamination and less water
vapor during nighttime.
μ
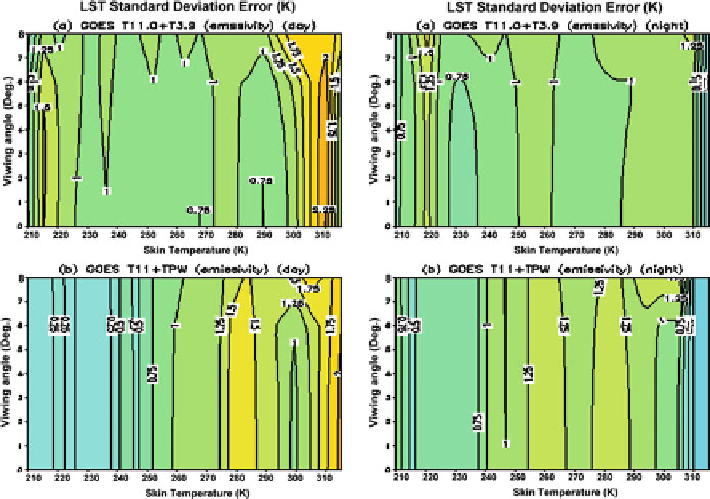
Search WWH ::

Custom Search