Information Technology Reference
In-Depth Information
Fig. 3.4
Visualization of the learned attribute relation compatibility (best viewed in color)
3.6.2 Experimental Results and Analysis
3.6.2.1 Attribute Relation Compatibility
Figure
3.4
illustrates the visualization of user attribute relation compatibility
ʨ(
. The size of the circle and line is proportional to the strength of
the relation compatibility. We see that the results of mined attribute relation compat-
ibility are reasonable, such as young with student, young with unmarried, elder with
married, male with politician, female with fun things, and positive with married. In
particular, personal occupation and interest exhibit strong correlations. For example,
IT person has strong relationship with technology and business; entertainer corre-
lates with entertainment and funny issues. These meaningful attribute relation pat-
terns derived from the Relational LSVMmodel suggest that when inferring attribute
“photographer” for a user or retrieving users with attribute “photographer”, the pres-
ence of interest attribute “photography” is a useful indicator to the relevant users.
Our relational SVM model incorporates the raw values of attribute relations to help
learn attribute inference and attribute relation compatibility. The model encourages
the helpful attribute pairs and suppresses the negative pairs. In this learning man-
ner, the true attribute pairs will be maintained. Hence the learned attribute relations
are accurate and useful for attribute inference. These results clearly demonstrate the
effectiveness of the proposed model in mining popular attribute relation patterns.
a
i
,
a
k
)
∈{
ʳ,ʷ
}
3.6.2.2 User Attribute Inference
To train a model for each type of attribute, we select the current type of attribute as
target attribute and the rest types as auxiliary attributes. We note that inferring user
interest attribute is a binary inference task. So we train a model for each value of user
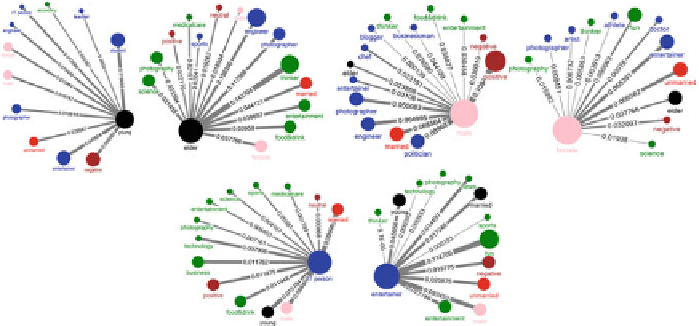