Information Technology Reference
In-Depth Information
0.8
0.6
0.4
0.2
0
−0.2
−0.4
−0.6
−0.8
10
5
10
5
0
0
y
−5
−5
x
−10
−10
Fig. 12.21
Approximation of the test function of Eq. (
12.27
), using an RBF neural network with
Gaussian basis functions
Table 12.1
Evaluation of
NN with Hermite basis
functions
Function
FNN type
Num nodes
RMSE
Hermite
6
0.613
Eq. (
12.23
)
OHL-FNN
6
0.187
RBF
6
0.223
Hermite
6
0.034
Eq. (
12.24
)
OHL-FNN
6
0.059
RBF
6
0.373
Hermite
6
0.726
Eq. (
12.25
)
OHL-FNN
6
0.339
RBF
6
1.565
Hermite
16
0.047
Eq. (
12.26
)
OHL-FNN
16
0.121
RBF
16
0.050
Hermite
16
0.032
Eq. (
12.27
)
OHL-FNN
16
0.117
RBF
16
0.028
12.7
Applications to Image Compression
The suitability of NN based on the QHO eigenstates for function approximation
and system modelling has been evaluated in [
164
], using the benchmark tests
given in [
221
]. Here, the image compression capabilities of FNN with Hermite
basis functions will be evaluated. Regarding the structure of FNN used for image
compression, the hidden layer has fewer nodes than the input layer, and the hidden
layer output values are considered as the compressed image (see Fig.
12.22
a). In
order to reconstruct the compressed image, the weights between the nodes of the
hidden and the output layer are also transmitted. If the number of the output values
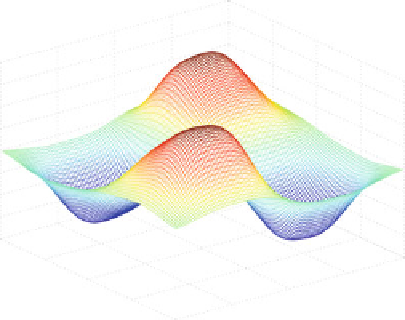

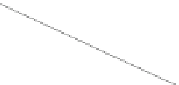



















