Information Technology Reference
In-Depth Information
Table 12.2
Probability density table for features type 1
Concept
(Probablity)
F1
F2
F3
F4
Inter
(0.48)
0.35
0.41
0.37
0.00
Periodic
(0.42)
0.30
0.27
0.30
0.00
Poly
(0.07)
0.29
0.24
0.33
0.00
Prime
(0.02)
0.03
0.03
0.00
0.00
Factorial
(0.01)
0.04
0.05
0.00
1.00
Fig. 12.4
Learning concepts
using features
Features 1
Features 2
Retroduction
Abstraction
Rank
Learning
Concepts
Result
Controller
Result
The initial probabilities of each hypothesis are either fixed to make each equally
probable or are calculated from a training set by using a trainer. The trainer keeps
past classifications of hypotheses and its features with its probability value in
two
tables
. These are:
•A
probability density-table
to keep track of the history of occurrences of a
hypothesis with the features of a sequence that produce such hypothesis,
•A
running probability table
to keep records of occurrences of each hypothesis.
Bayes rule provides the underlying mechanism for our classification. Both tables
have to be 'trained' using a training set to establish their probability values. The
effect of training is to accumulate experience and use them to “recognize” variations
to be used for our classification of hypotheses. Training data was provided from
standard IQ tests set by Eysenck (
1974a
,
b
). This data gives sequences from where
actual hypotheses and features were extracted and used as input for the training
session. Using these training patterns, we update the two probability density-table
using the rule

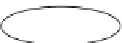
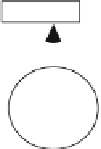




































































