Environmental Engineering Reference
In-Depth Information
ses and scenario analyses can generate huge quantities of data, and presenting these
data in a digestible form is an art. The first step is to have a robust metric that
captures the key question you wish to ask—for example, in Box 5.3, the metric is
the 'time to crisis'. Then you can use tables or graphs to show how the metric varies
between scenarios.
The main consideration in choosing a model is
parsimony
. The model should
be the simplest possible that allows you to answer the question. This is because
models are most effective when they are simple enough to provide an intuitive
understanding of the system, and all the unnecessary components are stripped
out so that the key drivers of the system can be explored without distraction.
Complex models have more parameters contributing to model error (both in
terms of debugging and in terms of the uncertainty inherent in parameter
estimation).
Deciding what the key drivers of system dynamics are is a matter of having
a really good understanding of the system, backed up by a thorough read of the
literature to see what people have done in similar systems. For example, is spatial
structure a key component of the system? Is it necessary to separate the prey
population into age, sex or size classes? Is it important to include stochasticity or
not? If you are unsure, a useful way to address the level of complexity required in a
model is to start with the more complex version of the model, and then
compare
its results
to those generated by a simpler version—is the behaviour of the simpler
model qualitatively different to that of the more complex and presumably more
realistic model? If not, choose the simpler model. People who have done this
kind of testing tend to find that simpler models are more robust and reliable than
complex ones. For example, Hilborn and Walters (1987) used a relatively complex
age-structured model to generate a dataset, and then used this dataset to test the
predictive power of both the same model and a simple lumped population model.
The simple model performed better, even though it hadn't been used to generate
the data. The simplest approach was also preferred in the competition run by the
International Whaling Commission to find a model for whale management—in
this case, a statistical model that fitted a curve to the empirical data out-performed
more complex mechanistic models (Box 7.7).
The best model to use depends both on the biology of the species and on the
management options you will be exploring. The main division when modelling
populations is between lumped and structured models, but there are several ways
in which a model can be structured (Table 5.2). Biological models can also be at the
genetic, community and ecosystem levels, but these are less commonly used for
modelling the sustainability of hunting.
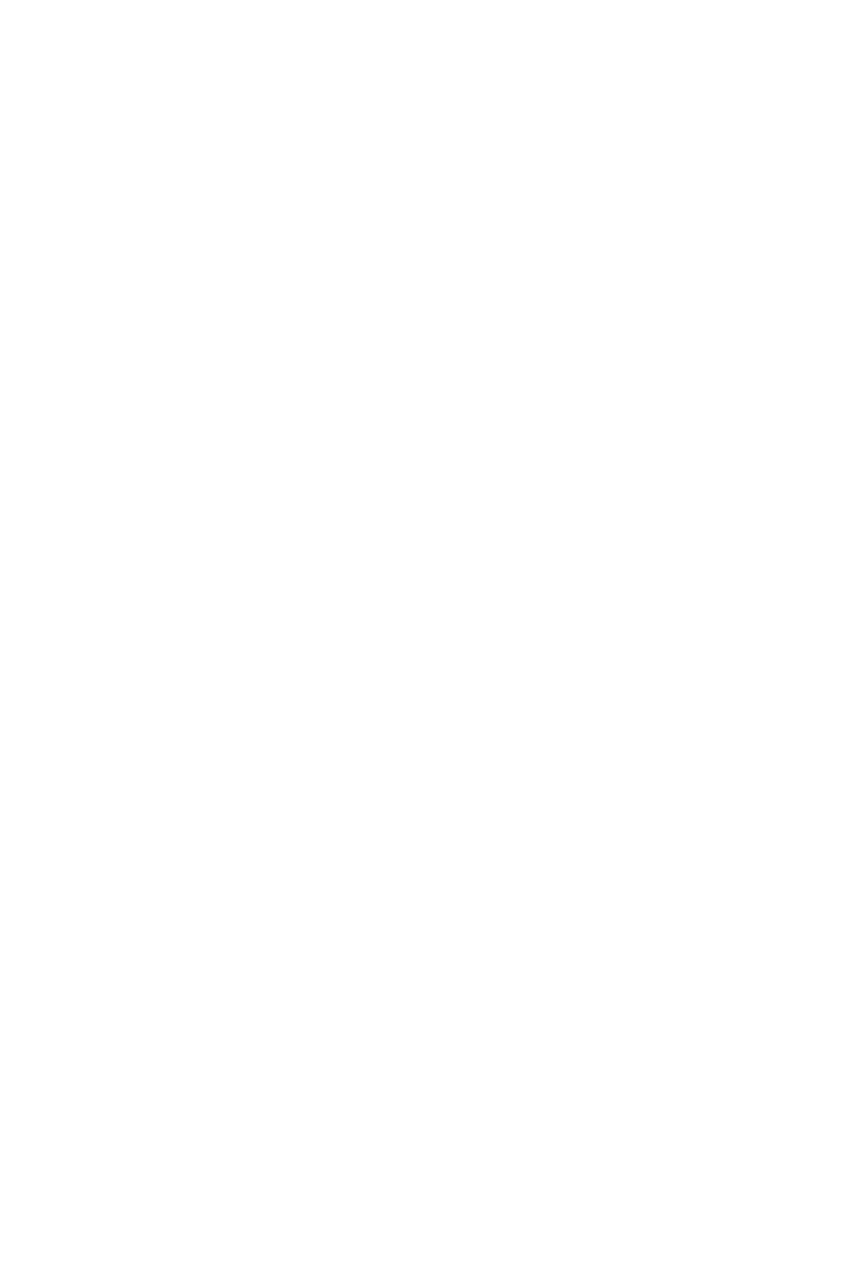