Information Technology Reference
In-Depth Information
Fig. 7.6
Spatial filters (i.e., weight attributed to each channel) obtained to classify
left hand
versus
right hand
motor imagery. The electrodes, represented by
black dots
, are here seen from
above
, with the subject nose on
top
. a basic CSP algorithm, b RCSP with a penalty term imposing
spatial smoothness, c RCSP with a penalty term penalizing unlikely channels according to EEG
data from other subjects
performed. For instance, this can be done manually (by trial and errors), or by
looking at the average EEG frequency spectrum in each class. In a more automatic
way, possible methods include extracting band-power features in multiple fre-
quency bands and then selecting the relevant ones using feature selection (Lotte
et al.
2010
), by computing statistics on the spectrum to identify the relevant fre-
quencies (Zhong et al.
2008
), or even by computing optimal band-pass
filters for
classi
cation (Devlaminck
2011
). These ideas can be used within the CSP frame-
work in order to optimize the use of both the spatial and spectral information.
Several variants of CSP have been proposed in order to optimize spatial and
spectral
filters at the same time (Lemm et al.
2005
; Dornhege et al.
2006
; Tomioka
et al.
2006
; Thomas et al.
2009
). A simple and computationally ef
cient method is
worth describing: The
filter bank CSP (FBCSP) (Ang et al.
2012
). This method,
illustrated in Fig.
7.7
, consists in
rst
filtering EEG signals in multiple frequency
bands using a
filters are opti-
mized using the classical CSP algorithm. Finally, among the multiple spatial
filter bank. Then, for each frequency band, spatial
filters
obtained, the best resulting features are selected using feature selection algorithms
(typically mutual information-based feature selection). As such, this selects both the
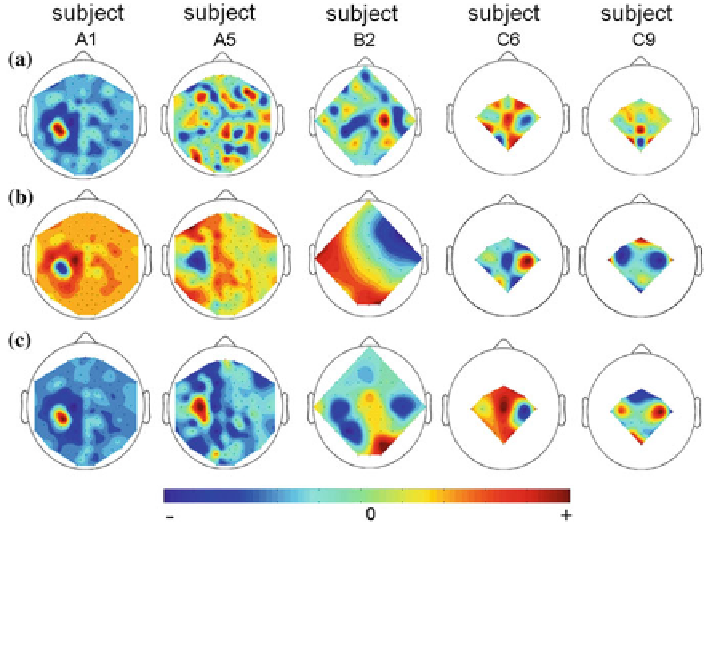