Information Technology Reference
In-Depth Information
Fig. 7.1
Simply put, data can be
big in the amount of measurements
on an individual (e.g., next
generation sequencing) or can be
big in the number of individuals on
whom there are some
measurements (e.g., clinical notes,
laboratory measurements, claims).
Naturally, it is exciting to imagine
what happens when we reach the
upper right quadrant
Next gen-seq,
iPOP
Claims,
EMR, Clinical notes
small
Large
Number of samples
companies (e.g., a typical social network profi le, when exported is a couple of
gigabytes) resulting in a gold rush around analyzing this “digital exhaust”
5
The idea of using data for enhancing health and well-being is popular in groups
such as the
Quantifi ed Self
collaborative and other self-tracking groups.
6
Given the
rising popularity of such efforts and the increasingly sophisticated collection of
phenotypic data enables “mass phenotyping,” which is the collection and integra-
tion of massive amounts of diverse phenotypical information (continuous or cate-
gorical variables) in order to discover latent patterns and correlate those patterns
with health and wellbeing [
12
].
In thinking about Big Data in healthcare—genomic, medical, environmental or
personal phenotypic—it is essential to think about the dimensions along which the
data are big. For example, genomic data are big in size but relatively small in num-
bers of samples; whereas claims data are small in size but are available for over 100
million individuals. Thinking along these two axes forms a sector-map (Fig.
7.1
),
which aids in thinking about potential analyses and computational solutions to use.
Finally, both disease and its treatment are processes that unfold over time. Hence,
it is essential to understand the nature and temporal density of any dataset that is
used. Continuous time-traces such as those collected by an electrocardiogram moni-
tor are very different from billing data, which are collected only when a person gets
sick and interacts with the health system. Similarly genomic data are usually a one-
time measurement and are rarely re-collected over time, except in highly special-
ized situations such as studying the response of a tumor to specifi c anti-neoplastic
drugs.
Depending on the axis along which the data are dense (samples, variables, and
time in Fig.
7.2
), different methods apply and lead to different insights. For exam-
ple, relatively simple methods based on recognizing mentions of drugs, diseases,
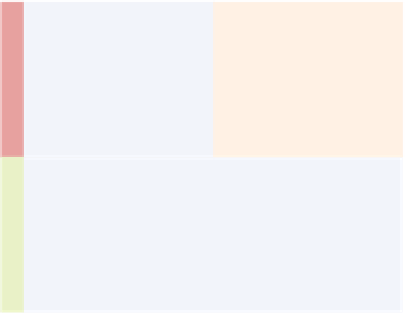











Search WWH ::

Custom Search