Information Technology Reference
In-Depth Information
2.
Generalized Estimating Equations
(
GEE
). Again, this approach
assumes asymptotic normality for conducting inference, a good
approximation only when the number of groups is large.
3.
Randomization-Based Inference
. Unequal-sized groups will
result in unequal variances of treatment means resulting in mis-
leading
p
values. To be fair, “Gail et al. [1996] demonstrate that
in GRTs, the permutation test remains valid (exact or near exact
in nominal levels) under almost all practical situations, including
unbalanced group sizes, as long as the number of groups are
equal between treatment arms or equal within each block if block-
ing is used.”
The drawbacks of all three methods, including randomization-based
inference if corrections are made for covariates, are the same as those for
other methods of regression as detailed in Chapters 8 and 9.
TABLE 5.2 Comparison of Different Analysis Methods for Inference on Treatment
Effect
b
ˆ
a
Method 10
2
b
ˆ
(10
2
SE)
p
Value
ˆ
Fruit /vegetable
GLIM (independent)
-
6.9 (2.0)
0.0006
GEE (exchangeable)
-
6.8 (2.4)
0.0052
0.0048
GLMM (random intercept)
-
6.7 (2.6)
0.023
0.0077
df D 12
b
Permutation
-
6.1 (3.4)
0.095
t
test (group level)
-
6.1 (3.4)
0.098
Permutation (residual)
-
6.3 (2.9)
0.052
Smoking
GLIM (independent)
-
7.8 (12)
0.53
GEE (exchangeable)
-
6.2 (20)
0.76
0.0185
GLMM (random intercept)
-
13 (21)
0.55
0.020
df D 12
b
Permutation
-
12 (27)
0.66
t
-test (group-level)
-
12 (27)
0.66
Permutation (residual)
-
13 (20)
0.53
a
Using Seattle 5-a-day data with 26 work sites (
K
=
13) and an average of 87 (
n
i
ranges
from 47 to 105) participants per work site. The dependent variables are ln (daily servings
of fruit and vegetable C
1
) and smoking status. The study design is matched pair, with
two cross-sectional surveys at baseline and 2-year follow-up. Pairs identification, work
sites nested within treatment, intervention indicator, and baseline work-site mean fruit-
and-vegetable intake are included in the model. Pairs and work sites are random effects
in GLMM (generalized linear mixed models). We used SAS PROC GENMOD for GLIM
(linear regression and generalized linear models) and GEE (generalized estimating
equations) (logistic model for smoking data) and SAS PROCMIXED (for fruit/vegetable
data) or GLMMIX (logistic regression for smoking data) for GLMM; permutation tests
(logit for smoking data) were programmed in SAS.
b
Degrees of freedom (df)
=
2245 in SAS output if work site is not defined as being
nested within treatment.
Source
: Reprinted with permission from the
Annual Review of Public Health
Volume 22,
© 2001 by Annual Reviews. Feng et al. [2002].
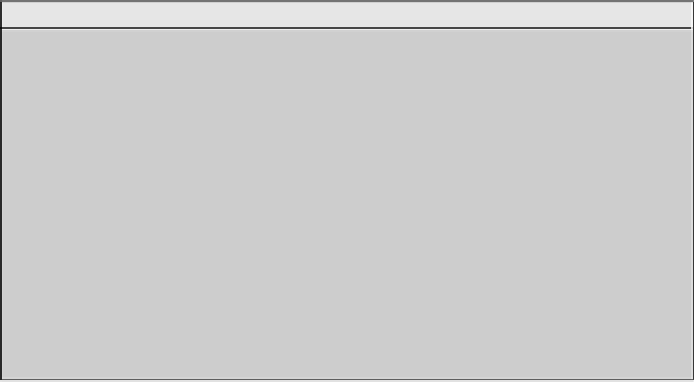
