Information Technology Reference
In-Depth Information
Its ARE relative to the mean is 0.97 when
F
is a normal distribution
(Lehmann, 1998, p. 246). With little to lose with respect to the mean
if
F
is near normal, and much to gain if
F
is not, the Hodges-Lehmann
estimator is recommended.
Suppose {
X
i
} and {
Y
j
} are i.i.d. with distributions Pr{
X
i
£
x
} =
F
[
x
]
and Pr {
Y
j
£
y
} =
F
[
y
-D] and we want to estimate the shift parameter D
without having to specify the form of the distribution
F
. For a normal
distribution
F
, the optimal estimator with least-square losses is
1
mn
Â
Â
(
)
=-
D=
Y
-
X
Y
X
,
j
i
i
j
the arithmetic average of the
mn
differences
Y
j
-
X
i
. Means are highly
dependent on extreme values; a more robust estimator is given by
ˆ
(
)
D=
median
ij
XX
-
.
j
i
Minimum Loss
The value taken by an estimate, its accuracy (that is, the degree to which
it comes close to the true value of the estimated parameter), and the asso-
ciated losses will vary from sample to sample.
A minimum loss estimator
is one that minimizes the losses when the losses are averaged over the set
of all possible samples. Thus its form depends upon all of the following:
the loss function, the population from which the sample is drawn, and
the population characteristic that is being estimated. An estimate that is
optimal in one situation may only exacerbate losses in another.
Minimum loss estimators in the case of least-square losses are widely
and well documented for a wide variety of cases. Linear regression with
an LAD loss function is discussed in Chapter 9.
Mini-Max Estimators
It's easy to envision situations in which we are less concerned with the
average loss than with the maximum possible loss we may incur by using a
particular estimation procedure. An estimate that minimizes the maximum
possible loss is termed a mini-max estimator. Alas, few off-the-shelf
mini-max solutions are available for practical cases, but see Pilz [1991]
and Pinelis [1988].
Other Estimation Criteria
The expected value of an
unbiased
estimator is the population
characteristic being estimated. Thus, unbiased estimators are also
consistent estimators.
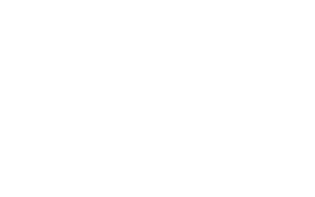

