Information Technology Reference
In-Depth Information
2
1
2
Ê
Ë
ˆ
¯
2
2
()()
=
()
-
()
vz
z
wz
exp
z
2
F
z
(9)
p
and
2
1
2
Ê
Á
ˆ
˜
(
)
()
-
()
=+
wz
z
3
z
F
z
z
2
exp
-
z
2
.
p
In particular, n is continuous. Intuition suggests that n be positive. This
fact will not be needed here, but it is true: see Diaconis and Freedman
(1982, (3.15)-(3.16)).
Proposition.
Assume (1) and (2). In probability:
q
n
,a
/
n
Æ ar and
R
n
,a
Æ
g
(l) and
Æ
()
-
()
-
g
l
a
1
g
lr
ar
F
.
(10)
n
,
a
1
In the second regression, the
t
statistic for testing whether a coefficient
vanishes is asymptotically distributed as
1
-
-
()
.
ar
lr
Z
l
1
g
These results may be interpreted as follows. The number of variables
in the first-pass regression is
p
= r
n
+
o
(
n
); the number in the second
pass is
q
n
,a
= ar
n
+
o
(
n
). That is, as may be expected, a of the variables
are significant at level a. Since
g
(l) < 1, the
R
2
in the second-pass
regression is essentially the fraction
g
(l) of
R
2
in the first pass. Likewise,
g
(l) > a, so the asymptotic value of the
F
statistic exceeds 1. Since the
number of degrees of freedom is growing, off-scale
P
values will result.
Finally, the real level of the
t
test may differ appreciably from the nominal
level.
Example.
Suppose
N
= 100 and
p
= 50, so r =
2
; and a = 0.25 so l
1.15. Then
g
(l)
2.9. In a regression with 50
explanatory variables and 100 data points, on the null hypothesis
R
2
should be nearly
0.72, and
E
{
Z
2
| |
Z
| > l}
2
.
Next, run the regression again, keeping only the variables significant at
the 25 percent level. The new
R
2
should be around
g
(l) = 72 percent of
the original
R
2
. The new
F
statistic should be around
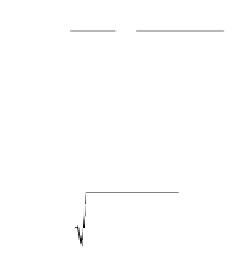









