Environmental Engineering Reference
In-Depth Information
to the following estimation variance of the kriged
value of
Y
n
∑
λ
j
C
(
x
−
x
)
=
C
(
x
−
x
),
i
=
1
,
…
(10.175)
,
n
i
j
i
j
=
1
n
∑
λ
Y Y
var
(
−
)
=
var
(
Y
)
−
C
(
x
−
x
)
(10.181)
i
i
This system of equations has only one solution if
C
(
h
) is a positive-definite function and
x
i
are distinct
locations (de Marsily, 1986). The weights
λ
i
derived from
the solution of Equations (10.175) correspond to the
mean-removed rSF
Y
(
x
); therefore, the original rSF is
estimated by the relation
i
=
1
which can be written in terms of the original variable,
Z
, as
n
∑
λ
Z Z
var
(
−
)
=
var
(
Z
)
−
C
(
x
−
x
)
(10.182)
i
i
n
∑
ˆ
i
=
1
Z
( )
x
= +
µ
λ
(
Z
−
µ
)
(10.176)
i
i
i
=
1
This equation is useful in calculating the distribution
of uncertainty in the kriged data, and can be used as a
measure of the accuracy of the interpolated data.
The basis for estimating the kriging weights is the
spatial covariance function,
C
(
h
), which is usually calcu-
lated from synoptic measurements using Equation
(10.167). In most cases, the calculated covariance func-
tion is fitted to an analytic covariance function that is
assumed to be isotropic and homogeneous in space.
Several commonly used analytic covariance functions
are listed in Table 10.11. The Gaussian, exponential and
spherical models have two parameters: the variance,
σ
2
,
and a length scale,
L
or
α
, that measures the distance
over which the random variable remains (spatially) cor-
related. The
range
is defined as the distance at which the
correlation is 0.05 (Kitanidis, 1993), and is directly
related to the length scale in the covariance function
(see Table 10.11). The Gaussian model is the only model
with parabolic behavior at the origin (
C
(
h
) ∼
h
2
), and is
typically used to represent a
regionalized variable
, which
where
μ
is the mean value of
Z
(
x
). Estimation of random
space functions using Equation (10.176) is called
simple
kriging
.
A useful feature of the kriging estimator is the capa-
bility to estimate the variance of the kriged value of the
rSF. This
estimation variance
is given by
2
ˆ
(
ˆ
ˆ
var(
Y Y
−
)
=
Y Y
−
)
−
Y Y
−
(10.177)
(
ˆ
(
ˆ
2
=
Y Y
−
)
2
−
Y Y
−
)
The second term on the right-hand side is equal to
zero since
n
∑
λ
1
ˆ
ˆ
(
Y Y
−
)
=
Y
−
Y
=
Y
−
Y
=
0
(10.178)
i
i
i
=
where 〈
Y
〉 and 〈
Y
i
〉 are equal to zero since the mean has
been subtracted from the original rSF,
Z
, to yield
Y
.
The estimation variance in Equation (10.177) can there-
fore be written as
TABLE 10.11. Commonly Used Stationary Covariance
Functions
Model
Expression
range
ˆ
(
ˆ
var(
Y Y
−
)
=
Y Y
−
)
2
(10.179)
h
L
2
Gaussian
C h
( )
=
σ
2
exp
−
1.75
L
2
The right-hand side of Equation (10.179) is given by
Equation (10.173) in terms of the station weights,
λ
i
, and
the covariance function,
C
(
h
). The values of
λ
i
are given
by the solutions to Equations (10.175), which can be
written in the form
h
L
3
L
Exponential
C h
( )
=
σ
2
exp
−
3
2
h
1
2
h
3
1
−
+
σ
2
for
0
≤ ≤
h
α
Spherical
C h
( ) =
α
α
3
α
0
for
h
>
α
n
n
n
∑
∑
∑
h
L
h
L
λλ
C
(
x
−
x
)
=
λ
C
(
x
−
x
)
(10.180)
Hole effect
C h
( )
=
σ
2
1
−
exp
−
-
i
j
i
j
i
i
i
=
1
j
=
1
i
=
1
0
h
h
>
=
0
0
nugget
effect
C h
( )
=
σδ
2
( )
h
=
-
Combining Equation (10.180) with Equation (10.173)
and substituting the result into Equation (10.179) leads
σ
2
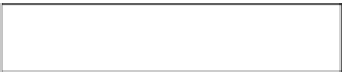
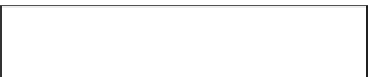
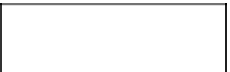




































Search WWH ::

Custom Search