Information Technology Reference
In-Depth Information
degraded
adaptive
iterative
degraded
original
thresholding
binarization
1
rejected
84 (37%)
0
average
recognition
error
0.3895
0.0190
0.75
0.5
original
adaptive
iterative
thresholding
binarization
rejected
0
0
0.25
average
recognition
error
0.0289
0.0195
0
0
0.25
0.5
0.75
1
(a)
(b)
Fig. 8.22.
Low-contrast Data Matrix test set recognition: (a) recognition error of iterative
binarization versus adaptive thresholding; (b) average recognition errors.
iterative binarization recognition error
8.7 Conclusions
In this chapter, it was shown that a non-trivial image processing task can be learned
by an instantiation of the Neural Abstraction Pyramid architecture. An adaptive
thresholding method was developed that is able to successfully binarize high-
contrast images of Data Matrix codes. Its results were used as desired output for
a Neural Abstraction Pyramid that was trained to iteratively produce them not only
for the original images, but also for degraded versions of them.
The network learns to recognize the cell structure of the Data Matrix and to
use it for binarization. The performance of both methods was evaluated using a
Data Matrix recognition system. The trained network performs as well as adaptive
thresholding for the original images, but outperforms it for degraded images. The
combination of both methods was able to lower the rejection rate significantly.
For the low-contrast red ink variant of the Data Matrix codes, the advantage of
iterative binarization is more obvious. It performs better than adaptive thresholding
for the original images and outperforms it dramatically for the degraded images.









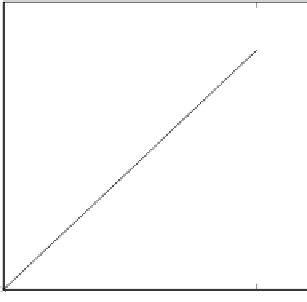



















































































Search WWH ::

Custom Search