Information Technology Reference
In-Depth Information
Contrasts
Input
ContrastSum
EdgeSum
1.75
0.58
1
2.05
0.78
2
2.28
0.97
3
2.24
0.96
4
2.25
0.78
5
2.22
0.63
6
2.09
0.64
7
5.24
3.12
8
E
kl
I
kl
Edge(
k
)
Excitatory Weights
Best Stimuli
Fig. 5.2.
Learning a hierarchy of sparse features - edge features. Shown are from left to right:
activity of the feature arrays for a digit (Input ”0”), excitatory weights to contrast features,
excitatory and inhibitory gain, stimuli that caused the highest winning activity. There are pairs
of step-edges for horizontal, vertical and (
/
) diagonal lines. The diagonal (
\
) is represented by
a single feature only. The feature in the last row detects background and reacts most strongly
to the inner part of small loops.
All learned edge features are different. There are pairs of horizontal, vertical, and
lower-left to upper-right diagonal step edges. The upper-left to lower-right diagonal
is represented by only one feature that responds to the center of the line. This is no
surprise since lines of this orientation are less frequent in handwriting. The feature
in the last row serves a special purpose. It is excited by centered background and
responds most to the inner part of small loops.
The edge features are not very specific. Since the inhibitory gain is small, they
respond also to suboptimal stimuli. Only the last feature is strongly inhibited by the
sum of the contrast features to avoid responses to foreground.
On the next higher level, Layer 2, the 16 features respond to lines of differ-
ent orientations. The line detectors show a sharper orientation tuning than the edge
features. Four line features that detect approximately horizontal lines are shown in




















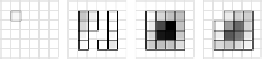





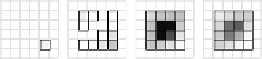


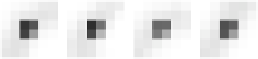


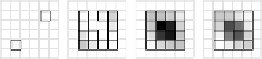


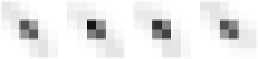











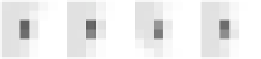





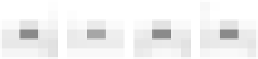











Search WWH ::

Custom Search