Information Technology Reference
In-Depth Information
Fig. 3.2.
Change of variables by PCA
Inertia on Centered and Reduced Data
For centered and reduced data, one has Tr
X
T
X
=
n.
Consider a sub-space of dimension
q<n
, and denote by
V
n×q
the matrix
R
q
; the scatter diagram projected on
R
q
is
associated with the projector on
represented by matrix
XV
, the inertia of which is
I
q
=Tr
V
T
X
T
XV
.
PCA defines the linear projection that maximizes
I
q
, the value of the inertia
of the points computed in
R
q
. That problem is solved by finding the first axis
with respect to which the inertia is maximum, then a second axis, orthogonal
to the previous one, to carry on with the maximization of the inertia, and
so on up to the
p
th axis. The axes obtained are borne by the eigenvectors of
matrix
X
T
X
, ranked in order of decreasing eigenvalues
λ
i
. The eigenvalues
λ
j
,
j
=1
,...,n
are positive or zero, since matrix
X
T
X
is positive symmetrical.
The transformation to be performed on of the centered data to obtain the
main components is
∈
R
n
→
V
T
n×q
∈
R
q<n
.
x
x
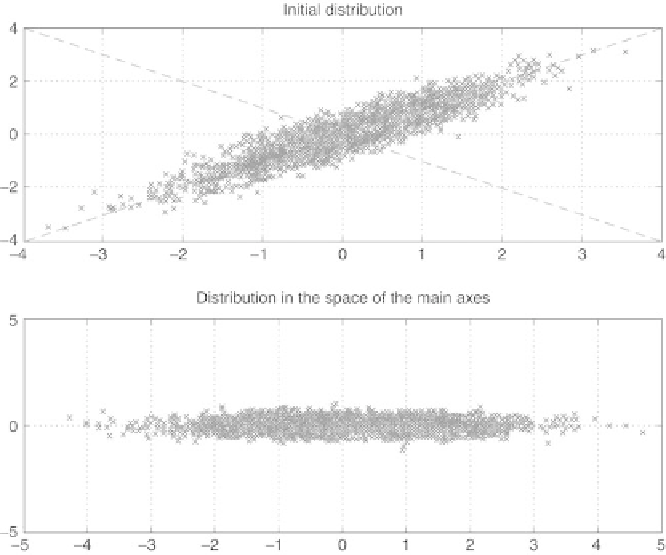
Search WWH ::

Custom Search