Information Technology Reference
In-Depth Information
Fig. 2.47.
Training and test sequences for the modeling of the hydraulic actuator
of a robot arm
around times 10 and 380) do not elicit responses whose amplitudes have the
same ratio.
No knowledge is available on the physics of the process, nor on any source
of disturbances. Therefore, assumptions on state noise and on output noise
must be tested.
Moreover, responses to stepwise inputs (for instance around time 220)
suggest that the order of the model should be larger than 1.
Finally, since the application does not require adaptive training, we con-
sider here batch training only.
2.7.4.1 Input-Output Model
Since input-output modeling is easier than state-space modeling, input-output
models are designed first. Since no prior knowledge about noise and distur-
bances is available, the state noise assumption (directed training of a feedfor-
ward model, NARX model), the output noise assumption (semidirected train-
ing of a recurrent model), and the output noise and state noise assumption
(training with both the model predictions and the process outputs present as
model inputs), are tested.
The assumptions involving state noise lead to very poor results when the
resulting models are operated as simulators, i.e., if they are asked to perform
predictions more than one-step ahead; they are not shown here. Semidirected
training of a recurrent model yields more satisfactory results. The best model
is a second-order model with three hidden neurons with sigmoid activation
function. Its equation is
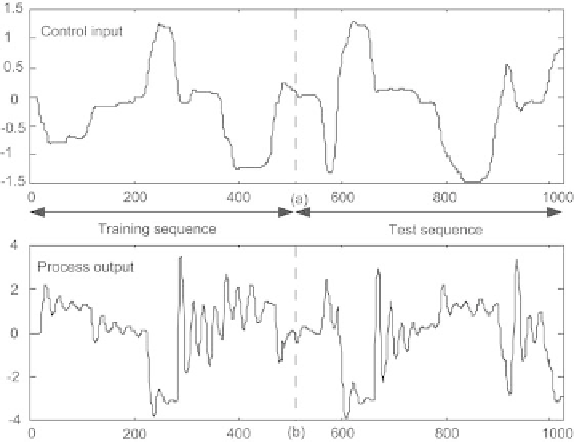
Search WWH ::

Custom Search