Image Processing Reference
In-Depth Information
14
0.45
B05
XCK
BCD−LS
EM−PPCA
EM−LDS
BCD−LS
EM−PPCA
EM−LDS
0.4
12
0.35
10
0.3
8
0.25
0.2
6
0.15
4
0.1
2
0.05
0
0
1
1.5
2
2.5
3
3.5
4
4.5
5
1
1.5
2
2.5
3
3.5
4
4.5
5
number of shapes (K)
number of shapes (K)
(a)
(b)
Figure 6.8:
Evaluation of the robustness to the number of basis shapes on the shark data for the algorithms
proposed in
Torresani
et al.
[
2008
] (BCD-LS, EM-PPCA, EM-LDS) and the methods in
Xiao
et al.
[
2004b
] (XCK) and in
Brand
[
2005
] (B05). The plot in (b) shows a zoomed version of the one in (a)
to highlight the differences between the
Torresani
et al.
[
2008
] algorithms. Courtesy of A. Hertzmann.
© 2008 IEEE.
In the same spirit of constraining the reconstruction of the shape basis, the notion of shape
priors was introduced in
Del Bue
[
2008
]. The general idea was to make use of known 3D shapes, and
assume that they were generated by part of the same shape basis as the deformation observed in the
input images. More specifically, let
L
be the 3
l
×
N
c
matrix of known 3D shapes. The factorization
problem of Eq.
6.1
can be re-written as
B
J
B
I
,
W
=[
C
J
|
C
I
]
(6.16)
to which can be added constraints for the shape prior as
L
=
NB
J
,
(6.17)
where
J
is the set of indices of the basis shapes that are common to the prior shapes and the unknown
ones, and
I
is the set containing the remaining indices. The factorization of both equations can then
be done simultaneously via generalized singular value decomposition. The shapes given as prior were
shown to significantly help reducing the ambiguities in the reconstructed shape basis, and, thus, in
the overall factorization.
Instead of adding explicit constraints on the shape coefficients, in
Torresani
et al.
[
2008
], it was
proposed to replace the linear subspace model with probabilistic PCA (PPCA)
Tipping and Bishop
[
1999
], and to introduce a Gaussian prior on the coefficients. A benefit of using PPCA is that
it makes it possible to marginalize out the shape coefficients. Therefore, the weights
c
k
are never





























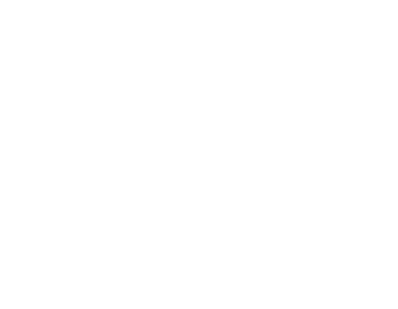





































































Search WWH ::

Custom Search