Biomedical Engineering Reference
In-Depth Information
Initialization
Segmentation without prior
Figure 4.
Segmentation without prior. Since there is no shape constraint imposed upon
the contour — other than a small length constraint present in the Chan-Vese model —
the boundary gradually separates brighter from darker areas. This indicates that intensity
information is insufficient to induce the desired segmentation.
Figure 5.
Boundary evolution for an ultrasound segmentation with uniform shape prior.
By constraining the level set evolution to the linear subspace spanned by the first few
eigenmodes computed from a set of training shapes, one can improve the segmentation of
the given image (see, e.g., [7]). Nevertheless, in our application, the uniform shape prior
does not sufficiently constrain the segmentation process, permitting the boundary to leak
into darker image areas.
Figure 6.
Boundary evolution for an ultrasound segmentation with nonparametric shape
prior. Imposing a non-parametric shape prior within the eigenspace spanned by the training
shapes leads to a segmentation process that is sufficiently constrained to enable an accurate
segmentation of the left ventricle. In contrast to the uniform prior (see Figure 5, the
nonparametric one does suppress leaking of the boundary), because it constrains the level
set function to a well-defined submanifold around the training shapes (see also Figure 2).
As a quantitative evaluation we computed the percentage of correctly clas-
sified object pixels and that of misclassified ones. During energy minimization,
the percentage of correctly classified pixels increases from 56 to 90%, while the
percentage of false positives decreases from 27 to 2.7% by using the kernel prior.
Using the uniform prior, we attain 92% correctly classified, yet the percentage of
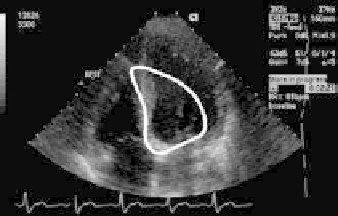



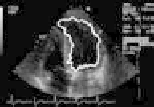


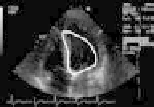
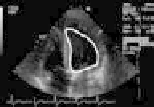
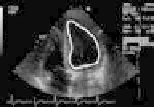