Biomedical Engineering Reference
In-Depth Information
(a)
(b)
(c)
(d)
Figure 18.
Segmentation of a polar IVUS image. Example using different filter spaces
and a combination of filters: (a) original image; (b) filter space for deformation using co-
occurrence matrix measures (IDM); (c) filter space for deformation using co-occurrence
matrix measures (Inertia); (d) filter space composed by the linear combination of IDM and
Inertia.
6.3. Applying STOP and GO to Medical Images
The following section includes several examples of STOP and GO active
models applied to different medical images and with different space maps.
Figure 18 shows an example of tissue detection on polar transformation of
intravascular ultrasound images using different filter spaces. Figure 18a shows
the original image, while Figure 18b shows the segmentation using the inverse
differential moment of the co-occurrence matrix at zero degrees, Figure 18c shows
the final snake using the inertia of the co-occurrence matrix, and Figure 18d depicts
the result using a linear combination of the inertia and inverse different moment.
Figure 19 shows the segmentation of turbid liquid in endoscopic images ac-
quired by a wireless capsule using a confidence rate space. We have trained a
40-round adaboost with decision stumps using general bubble data. Figure 19a
shows the original image, while Figure 19b displays the confidence rate of the
classifier. As we can observe, the confidence rate at the regions of interest is
higher than in rest of the areas. Figure 19c shows a geometric enhancement of the
confidence rate map, and Figure 19d displays the resulting segmentation.
Figure 20 shows another example of the segmentation of bubbles using a
confidence rate spaces. Using the same model and classifier, we built a 40-round
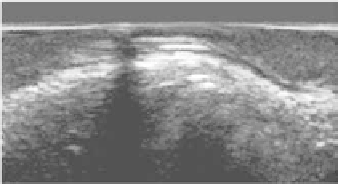

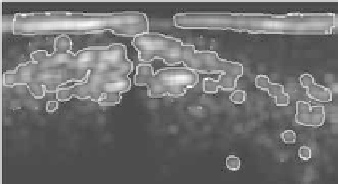
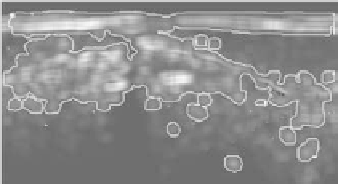