Information Technology Reference
In-Depth Information
and “Jump ball at the court center” events). A correct recognition rate of 89.8% was
here obtained with the HPaHMM. This result has to be compared with the 92.2%
correct recognition rate obtained with the HPaSMM method.
For a large majority of the 25 processed video segments, HPaHMM gave similar
segmentations than those obtained with the HPaSMM methods. However, for some
segments, the HPaHMM method provided a less accurate segmentation than the
HPaSMM method. Figure 17 illustrates these results. It presents results obtained by
both methods on the first segment of the processed handball video. For that segment,
correctness rate is 60% with HPaHMM method whereas the HPaSMM one reaches
89% correct recognition rate.
Fig. 17
Plot of the recognition results for the first segment of the video. Left: results obtained
with the HPaSMM method. Right: results obtained with the HPaHMM method. For both
methods, ground truth is plotted in red and obtained results are plotted in blue. Numbers
on the vertical axis correspond to activity phases numbering described in Section 4.2. The
horizontal axis denotes the numbering of the successive group of images (with parameter
k
group
value set to 8).
Such results highlight the advantage of modeling upper state durations since the
HPaSMM method obtains more efficient recognition results than the HPaHMM one.
First, this information enables to reduce time shifts that may appear at the transitions
between activity phases. Furthermore, as shown on Figure 17, this information also
helps HPaSMM method to avoid errors of activity recognition that may occur with
the HPaHMM method.
Computation time
For the conducted experiments, we used a Intel Pentium Centrino 1.86 GHz pro-
cessor.
k
group
was set to 8 (see Section 4.1). Computation times reported here cor-
respond to tests with the LOOCV procedure when omitting events “7-meter throw”
and “Jump ball at the court center”. Hence, training set of trajectories was com-
posed of 24 segments corresponding to approximately 8 minutes and 30 seconds
depending on the size of the segment left out and used for testing.
The computation time required to train the HPaSMM model was around 3 sec-
onds. Computation time for the recognition stage applied to one segment (the mean
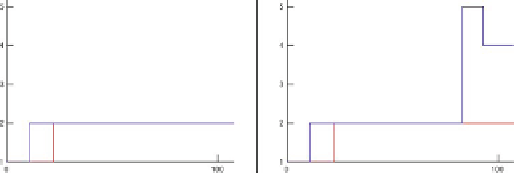



Search WWH ::

Custom Search