Information Technology Reference
In-Depth Information
Understanding Sports Video Using Players
Trajectories
Alexandre Hervieu and Patrick Bouthemy
Abstract.
One of the main goal for novel machine learning and computer vision sys-
tems is to perform automatic video event understanding. In this chapter, we present
a content-based approach for understanding sports videos using players trajectories.
To this aim, an object-based approach for temporal analysis of videos is described.
An original hierarchical parallel semi-Markov model (HPaSMM) is proposed. In
this latter, a lower level is used to model players trajectories motions and interactions
using parallel hidden Markov models, while an upper level relying on semi-Markov
chains is considered to describe activity phases. Such probabilistic graphical mod-
els help taking into account low level temporal causalities of trajectories features as
well as upper level temporal transitions between activity phases. Hence, it provides
an efficient and extensible machine learning tool for applications of sports video
semantic-based understanding such that segmentation, summarization and index-
ing. To illustrate the efficiency of the proposed modeling, application of the novel
modeling to two sports, and the corresponding results, are reported.
1
Introduction
Semantic-based interpretation of videos mainly consists in recognizing objects be-
haviors, where a behavior can be defined as a sequence of activities. Hence, the issue

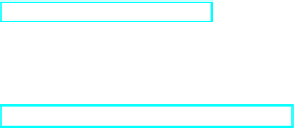
Search WWH ::

Custom Search