Information Technology Reference
In-Depth Information
distribution with
ν
degrees of freedom, (7.32) can be interpreted as incrementing
the degrees of freedom from an initial 2
a
τ
by
n
r
nk
. Thus, while the prior has
the weight of 2
a
τ
observations, each added observation is weighted according to
the responsibility that classifier
k
has for it. By using (7.30) and the relation
w
kj
T
x
n
)
2
r
nk
(
y
nj
−
n
r
nk
x
n
y
nj
+
w
kj
T
n
w
kj
,
=
n
2
w
kj
T
n
r
nk
y
nj
−
r
nk
x
n
x
n
Equation (7.33) can be reformulated to give
⎛
⎝
n
2
⎞
E
α
(
α
k
)
1
2
D
Y
⎠
.
b
τ
k
=
b
τ
+
W
k
x
n
2
+
w
kj
r
nk
y
n
−
j
(7.35)
This shows that
b
τ
is updated by the responsibility-weighted sum of squared
prediction errors, averaged over the different elements of the output vector, and
the average size of the
w
kj
's, weighted by the expectation of the weight precision
prior. Considering that
(Gam(
a, b
)) =
a/b
[19], the mean of the noise variance
posterior is therefore strongly influenced by the responsibility-weighted averaged
squared prediction error, given a suciently uninformative prior.
E
Classifier Weight Priors
q
α
7.3.3
(
α
)
As by (7.17),
p
(
α
) factorises with respect to
k
, we can treat the variational pos-
terior
q
α
for each classifier separately. For classifier
k
, this posterior is according
to (7.15), (7.16), (7.17) and (7.24) given by
ln
q
α
(
α
k
)=
α
k
)) + ln
p
(
α
k
) + const. (7.36)
Using (7.8), the expectation of weights and noise precision evaluates to
E
W,τ
(ln
p
(
W
k
,τ
k
|
E
W,τ
(ln
p
(
W
k
,τ
k
|
α
k
))
=
j
E
W,τ
ln
a
τ
,b
τ
)
(
w
kj
|
0
,
(
α
k
τ
k
)
−
1
I
)+lnGam(
τ
k
|
N
D
2
2
E
W,τ
(
τ
k
w
kj
w
kj
)
+ const.
=
j
α
k
ln
α
k
−
(7.37)
Also, by (7.9),
ln
p
(
α
k
)=(
a
α
−
1) ln
α
k
−
b
α
α
k
+ const.
(7.38)
Together, that gives the variational posterior
ln
q
α
(
α
k
)=
D
X
D
Y
2
1
ln
α
k
+
a
α
−
⎛
⎝
b
α
+
1
⎞
2
j
E
W,τ
(
τ
k
w
kj
w
kj
)
⎠
α
k
+const.
−
a
α
k
,b
α
k
)
,
=lnGam(
α
k
|
(7.39)





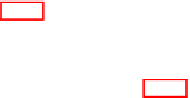




Search WWH ::

Custom Search