Information Technology Reference
In-Depth Information
c
3
c
2
c
1
Input Space
Fig. 5.3.
Classification data of two different classes and three classifiers. The circles
represent class 1, and the squares are samples of class 2. The larger rectangles within
the input space are the matched ares of the three classifiers
c
1
,
c
2
,and
c
3
.
Table 5.2.
Estimates resulting from the classification task illustrated in Fig. 5.3. The
table gives the number of observations of each class matched per classifier. Additionally,
it shows the parameter estimate
w
and the measure
τ
−
1
of each classifier's prediction
quality, evaluated by (5.82) and (5.78) respectively.
w
k
τ
−
1
k
Classifier Class 1 Class 2
c
1
27
5
(0
.
84
,
0
.
16) 0
.
134
c
2
7
10
(0
.
41
,
0
.
59) 0
.
242
c
3
2
19
(0
.
09
,
0
.
91) 0
.
082
5.6
Discussion and Summary
The aim of a local model representing a classifier is to maximise its likelihood,
as follows from the probabilistic LCS model of the previous chapter. In this
chapter, several batch and incremental learning approaches for training linear
regression models and classification models have been described and compared.
With respect to linear regression, the maximum likelihood estimate of the
weight vector was shown to be a weighted least squares problem (5.5), that by
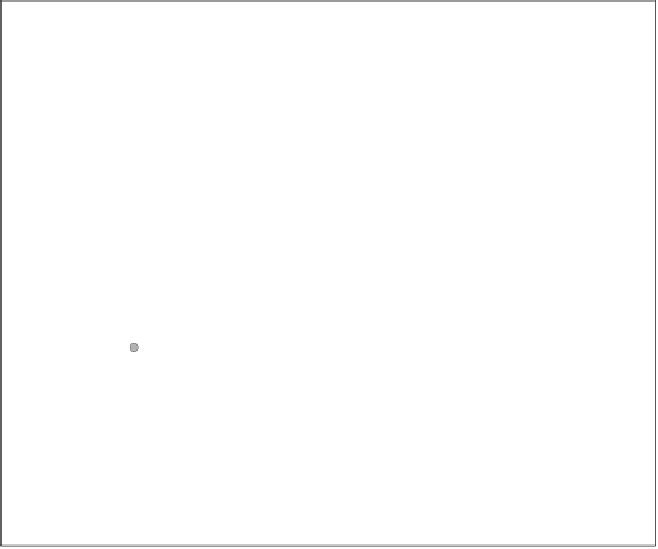














































































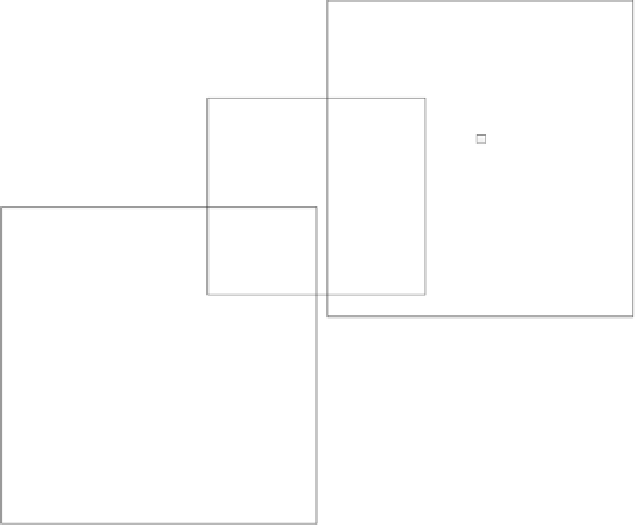





Search WWH ::

Custom Search