Information Technology Reference
In-Depth Information
As can be seen rendering speed decreases with size of rendered object but not nec-
essarily with resolution of the screen. In example for model 1 with no semi trans-
parent pixels for 1280 x 768 we have 49 fps, for 1152 x 864 43 fps and for 1280 x
800 47 fps. This is because model in 1152 x 864 is bigger (visualized with higher
number of pixels) then in two other cases.
For author's hardware the best configuration of application window size / cam-
era resolution and capture speed is 1024 x 768 (or 1280 x 768) for window 320 x
240 (30 fps) for camera captured image size or 1280 x 1024 for 640 x 480 (15
fps). In those two configurations all detected markers position will be instantly
computed to proper linear transformation of virtual 3D model and rendered.
The speed of rendering is higher for models with no semi transparent pictures
because they required less computation time in the shaders (GPU processors). The
example visualization performed by author's solution is presented in Fig 11.
Fig. 11
Example augmented reality visualization of three different models constructed from
CT data. From left to right: visualization of dense tissues (bones), model without semi
transparent pixels, model with semi transparent pixels for tissues with low density.
8 Summary
In this chapter the author had presented application of pattern classification me-
thods in the task of automatic interpretation of dynamic PCT maps. The process of
identification is consisted of three steps that utilize different pattern classification
approach. Firstly author uses heuristic algorithm that decides if the considered
perfusion map consists perfusion abnormality and determines its position (image
processing and abnormality detection). In the second step brain tissues are labeled
with brain AA with FFD algorithm. The last step is distinguishing between normal
and abnormal perfusion regions that might be detected in both hemispheres and
classification abnormal region to ischemic or hemorrhagic class. This classification
task is based on prior gathered medical knowledge. The author also presents the
test result of the proposed solution that was performed on real diagnostic data.
Advanced pattern classification methods can also be utilized in the process of
the AR visualization of medical data. Tracking algorithm detects the presence of
markers, determines its type with template matching algorithm and measures its
relation to camera (translation and rotation). Author describes one of many AR
solutions that can be easily attached to nearly all medical and non medical applica-
tion. AR gives support of a new level by presenting more informative and realistic
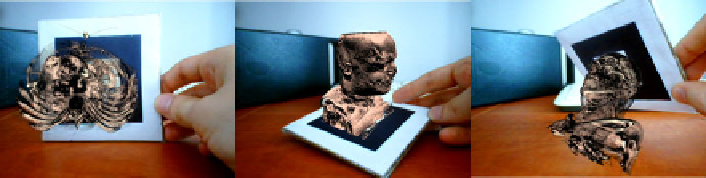
Search WWH ::

Custom Search