Database Reference
In-Depth Information
Figure 5.5
Indicative data setup for behavioral segmentations.
4.
Data validation and cleaning:
A critical issue for the success of any data
mining project is the validity of the used data. The data exploration and
validation process includes the use of simple descriptive statistics and charts
for the identification of inconsistencies, errors, missing values, and outlier
(abnormal) cases. Outliers are cases that do not conform to the patterns of
''normal'' data. Various statistical techniques can be used in order to fill in
(impute) missing or outlier values. Outlier cases in particular require extra care.
Clustering algorithms are very sensitive to outliers since they tend to dominate
and distort the final solution. For general purpose behavioral segmentations,
the outlier cases can also be filtered out so that the effect of ''noisy'' records in
the formation of the clusters is minimized.
Problematic values, particularly demographic information, can also be
imputed or replaced by using external data, provided of course the external data
are legal, reliable, and can be linked to the internal data sources (e.g., through
the VAT number, post code, phone number, etc.).
5.
Data transformations and enrichment:
This phase deals with the enrich-
ment of the modeling dataset with derived fields such as ratios, percentages,
averages, and so on. The derived fields are typically created by the application
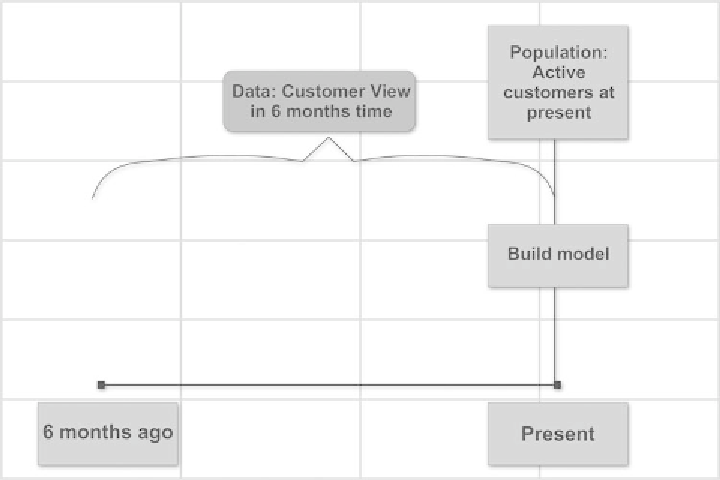
Search WWH ::

Custom Search