Information Technology Reference
In-Depth Information
set to a Gamma distribution with shape parameter
α
c
and scale parameter
b
c
.
m
c
,λ
0
k
,α
c
,b
c
,c
η
cc
,c
∈N
The quantities
are hyperparameters
to be specified. For this choice, we get valid joint Markov models for the
μ
k
's (and
therefore for the
θ
k
's) which are known as auto-normal [18] models. Whereas for
the standard Normal-Gamma conjugate prior the resulting conditional densities
fail in defining a proper joint model and caution must be exercised.
Standard Bayesian computations lead to a decomposition of (10) into two
maximizations: for
μ
k
, the product in (10) has a Gaussian form and the mode
is given by its mean. For
λ
k
, the product turns into a Gamma distribution and
its mode is given by the ratio of its shape parameter over its scale parameter.
After some straightforward algebra, we get the following updating formulas:
{
∈C}
and
{
(
c
)
}
c
i∈V
c
a
ik
y
i
+
λ
0
k
(
m
c
+
c
∈N
(
c
)
η
cc
(
μ
(
ν
)
k
=
λ
(
ν
)
k
− m
c
))
c
c
c
μ
(
ν
+1)
k
c
(11)
i∈V
c
a
ik
+
λ
0
k
λ
(
ν
)
k
c
c
α
c
+
i∈V
c
a
ik
/
2
−
1
b
c
+1
/
2[
i∈V
c
a
ik
(
y
i
− μ
(
ν
+1)
k
λ
(
ν
+1)
k
c
and
=
(12)
)
2
]
c
In these equations, quantities similar to the ones computed in standard EM for
the mean and variance parameters appear weighted with other terms due to
neighbors information. Namely, standard EM on voxels of
V
c
would estimate
μ
c
as
i∈V
c
a
ik
y
i
/
i∈V
c
a
ik
and
λ
c
as
i∈V
c
a
ik
/
i∈V
c
a
ik
(
y
i
−
μ
c
)
2
.Inthatsense
formulas (11) and (12) intrinsically encode cooperations between local models.
From these parameters values constant over subvolumes we compute parame-
ter values per voxel by using cubic splines interpolation between
θ
c
and
θ
c
for all
c
∈N
(
c
). We go back this way to our general setting which has the advantage
to ensure smooth variation between neighboring subvolumes and to intrinsically
handle nonuniformity of intensity inside each subvolume.
The key-point emphasized by these last derivations of our E and M steps
is that it is possible to go from a joint cooperative model to an alternating
procedure in which each step reduces to an intuitive well identified task. The
goal of the above developments was to propose a well based strategy to reach
such derivations. When cooperation exists, intuition is that it should be possible
to specify stages where each variable of interest is considered in turn but in a
way that uses the other variables current information. Interpretation is easier
because in each such stage the central part is played by one of the variable at a
time. Inference is facilitated because each step can be recast into a well identified
(Hidden MRF) setting for which a number of estimation techniques are available.
6R su s
We choose not to estimate the parameters
η
T
and
η
S
but fixed them to the
inverse of a decreasing temperature as proposed in [17]. In expressions (11) and
(12), we considered a general case but it is natural and common to simplify the


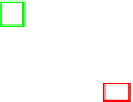




Search WWH ::

Custom Search