Biology Reference
In-Depth Information
As described above, well-curated metabolic network databases
[12,13] form the basis for any more detailed metabolic network
reconstruction or modeling task. For regulatory networks, similar
comprehensive databases covering genome-scale regulatory networks
in multiple organisms do not currently exist. The lack of databases
can be primarily attributed to the low degree of direct molecular level
conservation of regulatory network components (transcription factors
and especially binding sites) between distantly related species [77].
However, for individual organisms such network databases containing
experimentally verified regulatory interactions have been established,
the most prominent one being RegulonDB for
E. coli
[78]. In addition
to databases describing regulatory network structures, there are also
comprehensive databases specializing in describing transcription
factor (TF) binding sites such as TRANSFAC [79]. Although these
databases contain valuable information for regulatory network recon-
struction, they are not complete and for most part lack information
about the synergistic effects between TFs acting on one gene.
Nevertheless, these databases and primary research literature can be
used as a starting point to reconstruct regulatory networks for
well-characterized organisms such as
E. coli
based on genetic and
biochemical information reported in the primary literature [57,80].
The major advantage that regulatory network reconstruction has over
metabolic network reconstruction is the availability of high-throughput
experimental data directly relevant to the network structure. Data on
both network structure and network states in the form of genome-wide
promoter occupancy and mRNA expression data are widely available
for many commonly studied model organisms. Gene expression data
can be readily generated for well-studied organisms using a number
of standard technologies [81]. Advances in statistical data analysis
allow both establishing significant changes in gene expression between
conditions [82,83] and derivation of hypotheses about regulatory inter-
actions or coregulated gene modules directly from the data [84-86].
In particular, gene expression changes in response to deletions or
overexpression of regulatory genes have been productively used to
obtain sets of potential target genes for many regulatory proteins,
for example, in yeast and
E. coli
[57,87-90].
The development of the ChIP-chip (chromatin immunoprecipita-
tion followed by microarray analysis) method [91,92], which allows
directly detecting genomic target sites for DNA-binding proteins
such as TFs, has resulted in even more significant improvement in our
ability to reconstruct regulatory network structures. So far ChIP-chip
has been most extensively applied in yeast, where it has been used to
map the target genes of 203 TFs under a limited number of environ-
mental conditions [93,94], but in principle the technique can be readily
extended to other organisms [95,96]. ChIP-chip has also been applied
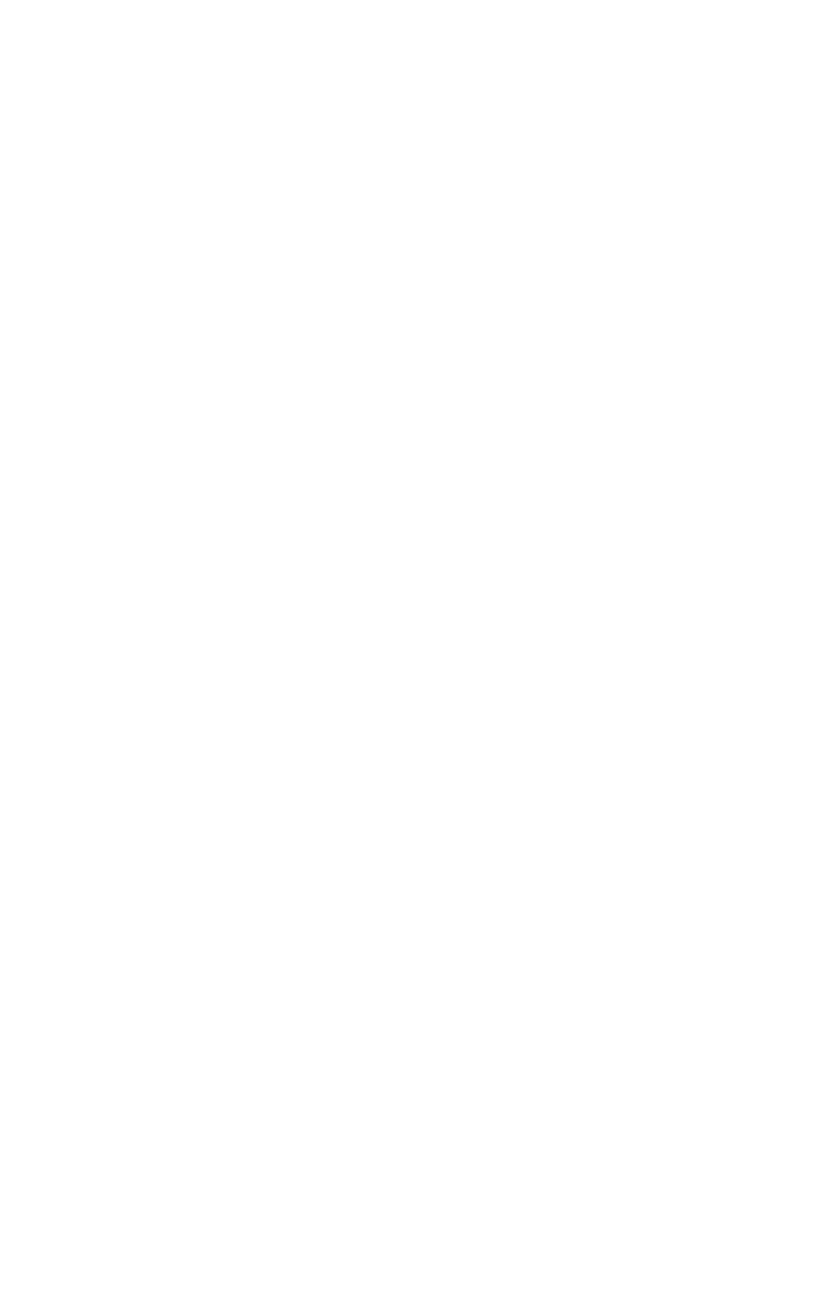
Search WWH ::

Custom Search