Biology Reference
In-Depth Information
by 4.6- and 37-fold, respectively. Based on these findings, strategies
for genome-scale metabolic engineering of
M. succiniciproducens
could
be suggested. It should be emphasized that genome-scale metabolic
flux analyses made it possible to understand previously unknown
metabolic characteristics from a minimal number of cultivation exper-
iments, which provided constraints for more accurate flux calculation.
In another study, combined analyses of the transcriptome and
metabolome were carried out for enhanced production of secondary
metabolite. Askenazi et al. [16] developed an
Aspergillus
strain over-
producing lovastatin, a cholesterol-lowering drug, based on these
analyses. Initially, the genes thought either to be involved in lovastatin
synthesis or known to broadly affect secondary metabolites production
in the parental strain were expressed to generate a library of strains.
Transcriptome profilings were carried out for these strains, followed
by a statistical association analysis to extract potential key parameters
affecting the production of lovastatin. From these results, the target
genes were identified and manipulated to improve lovastatin production
by over 50%. There is no doubt that more successful examples of employ-
ing combined x-omic analyses for strain improvement will appear.
IN SILICO MODELING AND SIMULATION
Several approaches have been developed for quantitative in silico mod-
eling and simulation of metabolic systems [20,40,41]. They can mainly
be classified into two types: kinetic model-based dynamic analysis and
constraints-based stationary analysis.
Kinetic Model-Based Analysis
In the dynamic approaches, kinetic and regulatory information is
incorporated into a kinetic model set of continuous differential equa-
tions. The mass conservation around metabolites is derived from the
detailed mechanisms and characteristics of the system; the resultant
rate equations can be formulated as:
dc
dt
(1)
=−
Sv
b
where
c
∈ ℜ
m
×
1
is the vector of the concentrations of
m
metabolites;
v
1
, the
n
-dimensional rate vector, whose
j
th component represents
the rate of reaction
j
as a nonlinear function of the concentrations of
the metabolites involved in the reaction,
v
j
=
∈ℜ
n
×
n
, the stoichio-
metric matrix of the network; and
b
is the vector of the net transport of
metabolites into and out of the cell and dilution due to cell growth.
Thus, this kinetic model can completely describe the dynamic behavior
as well as the structure of the metabolic network.
f
(
c
);
S
∈ ℜ
m
×
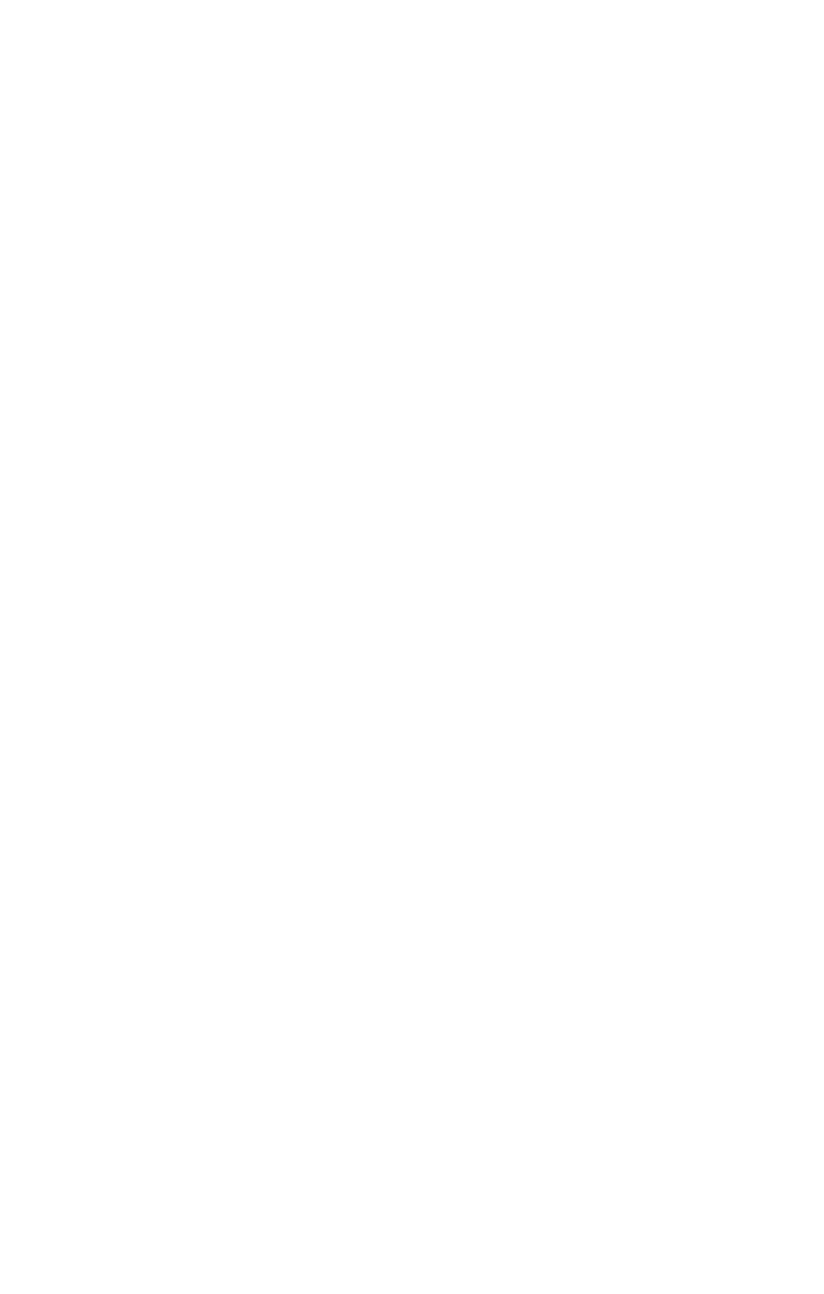
Search WWH ::

Custom Search