Information Technology Reference
In-Depth Information
∂
g
(
x
)
=
k
(
x
,
z
)
ij
z
=
x
∂
x
∂
z
i
j
2
x
−
z
k
(
x
,
z
)
=
exp{
},
In particular for the Gaussian kernel function
we have
2
2
σ
1
( =
.
In order to improve the performance of a SVM classifier, Amari and Wu
proposed a method of modifying a kernel function. To increase the margin or
separability of classes, we need to enlarge the spatial resolution around the
boundary surface in
g
x
δ
ij
ij
2
σ
U
. Let
c
(
x
) be a positive real differentiable function,
k
(
x,z
)
denote Gaussian kernel function, then
~
k
(
x
,
z
)
=
c
(
x
)
k
(
x
,
z
)
c
(
z
)
(8.42)
Also is a kernel function, and
~
2
g
(
x
)
=
c
(
x
)
c
(
x
)
+
c
(
x
)
g
ij
i
j
ij
∂
c
(
x
)
=
c
(
x
)
Where,
. Amari and Wu defined
c
(
x
) as
i
∂
x
i
2
x
−
x
i
Ã
2
c
(
x
)
=
h
i
e
2
τ
(8.43)
x
∈
SV
i
Where,
τ
is a positive number, hi denotes coefficient. Around the support vector
x
i
, we have
2
nr
2
h
σ
~
2
i
2
γ
2
g
(
x
)
≈
e
1
+
γ
σ
n
τ
4
τ
=
x
−
x
Where
is the Euclid distance between
x
and
x
i
. In order to make sure
i
~
g
(
x
)
is larger near the support vector
x
i
and is smaller in other region, we
need
σ
τ ≈
(8.44)
n
In summary, the training process of the new method consists of the two steps:
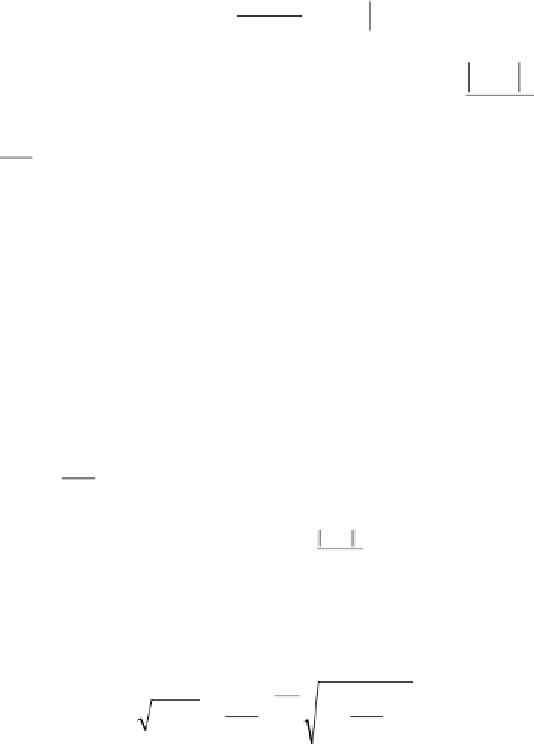

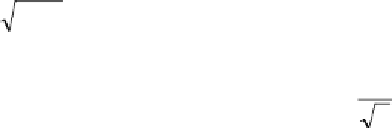