Information Technology Reference
In-Depth Information
and empirical risk. Meanwhile, it reflects risk bring structure complexity. There
are some relations between the confidence interval, VC dimension h and sample
number
l
. The inequality (8.14) could simply be expressed as:
R w
(
)
≤
R
(
w
)
+
(
h l
/ )
Φ
(8.14)
emp
According to the second term on the right-hand side of the inequality (8.14),
(
Φ
h l
/ )
increases. Therefore, the
confidence interval increases while learning machine's complexity and the VC
dimension increase. Moreover, the difference between actual risk and empirical
risk increase when learning machines use a small sample of training instances.
Note that the bound for the generalization ability of learning machines is a
conclusion in the worst case. Furthermore, the bound is not tight in many cases,
especially when VC dimension is higher. When
increases while the VC dimension,
h
h l
/
>0.37, the bound is
guaranteed not tight (Burges, 1998). When VC dimension is infinite, the bound
does not exist.
To construct small sample size methods we use both the bounds for the
generalization ability of learning machines with sets of totally bounded
nonnegative functions, 0
≤
L
w
∈Λ
(
Λ
is abstract parameters set), Each
bound is valid with probability at least 1-
η
.
(
z,w
)
≤
B,
4
R
(
w
B
ε
emp
l
(
)
≤
(
)
+
(
+
1
+
),
R
w
R
w
(8.15)
l
emp
2
B
ε
and the bound for the generalization ability of learning machines with sets of
unbounded functions,
R
(
w
emp
l
R
(
w
)
≤
(8.16)
l
1
−
a
(
p
)
τ
ε
)
+
1
p
−
1
p
−
1
a
(
p
)
=
(
)
p
2
p
−
2
where
ln
N
−
ln
η
ε
=
2
l
There are two methods to minimize the actual risk. One is to minimize the
empirical risk. According to upper formula, the upper bound of actual risk
decreases while the empirical risk decreases. The other is to minimize the second
term on the right-hand side of the inequality (8.15). We have to make the VC
dimension a controlling variable. Latter method conformances small sample size.
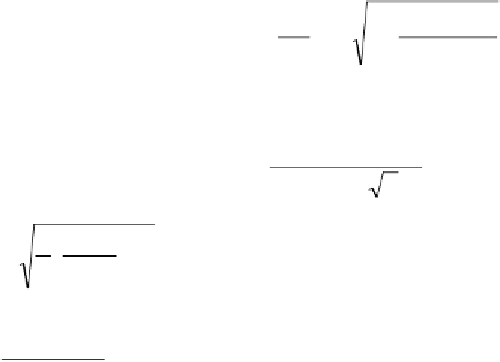