Information Technology Reference
In-Depth Information
among these variables. Data are described by the sufficient statistics in Table 6.3.
In this table, each entry represents a statistic of a state which cycles through all
possible configurations. For example, the first entry indicates that the statistic for
the configuration (SEX=male, SES=low, IQ=low, PE=low, CP=yes) is 4; the
second entry states that the statistic for the configuration (SEX=male, SES=low,
IQ=low, PE=low, CP=no) is 349. In the cycling of configuration of variables in
the table, the last variable (CP) varies most quickly, and then PE, IQ, SES. SEX
varies most slowly. Thus, the upper 4 lines are the statistics for male students and
the lower 4 lines are that of female students.
SEX
SEX
IQ
PE
SES
IQ
PE
SES
CP
CP
h
h
S
1
)
-45653
S
2
)
-45699
Log
p
(
D
|
Log
p
(
D
|
h
h
S
1
S
2
|
D
)
1.2×10
-10
p
(
|
D
)
1.0
p
(
Figure 6.1. Most likely network structures with no hidden variables
When analyzing data, we assume that there are no hidden variables. To
generate priors for network parameters, we utilize an equivalent sample size of 5
and a prior network where
h
c
S
) is uniform. Except that we exclude the
structure where SEX and/or SES have parents and/or CP has children, we assume
that all network structures are equally likely. Because the data set is complete, we
use formula (6.40) and (6.41) to calculate the posterior of network structure.
After searching all network structure exhaustively, we find two most likely
networks, which are shown in Figure 6.1. Note that the posterior probabilities of
the two most likely network structures are very close. If we adopt casual Markov
assumption and assume that there are no hidden variables, the arcs in the two
graphs can all be interpreted casually. Some of these results, such as the
influence of socioeconomic status and IQ to the college plan, are not surprising.
Some other results are very interesting: from both graphs, we can see that the
p
(
X
|





















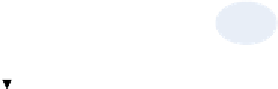




















