Information Technology Reference
In-Depth Information
can we get posterior increasingly close to the reality? We study this problem in
the following:
Let new sample
2
σ
x
1
, x
2
, … , x
n
is from normal distribution N(
,
), where
2
2
σ
x
d
is known and
ȶ
is unknown. If we use previous posterior h(
|
)= N(
1
,
)
1
x
as the prior of next round computation, then the new posterior is h
1
(
|
)=
2
2
2
d
N(
2
,
), where
1
n
1
n
1
n
n
x
Ã
=
2
2
−
1
i
n
α
=
(
α
+
x
)
/(
+
)
d
=
(
+
)
x
=
2
2
1
2
d
2
1
2
2
d
2
1
2
2
d
2
1
2
2
σ
σ
σ
.
i
1
1
n
1
n
α
=
(
µ
+
x
)
/(
+
)
We use
, the expectation of posterior
0
σ
2
0
σ
2
1
σ
2
0
σ
2
1
1
n
σ
2
1
x
α
=
(
µ
+
x
)
⋅
d
h
1
(
ȶ
|
) as the estimation of
ȶ
. Because
, we have
1
2
0
σ
2
0
2
1
1
n
1
n
σ
n
σ
α
=
2
2
2
2
(
α +
x
)
⋅
d
(
µ
+
x
2
x
⋅
d
=
+
)
2
2
1
1
0
2
d
2
1
σ
2
2
σ
2
0
2
1
2
1
n
σ
n
σ
2
2
2
2
(
µ
+
x
)
⋅
d
2
x
⋅
d
=
+
(6.9)
1
0
2
2
0
2
1
2
σ
n
1
n
1
n
n
1
n
2
2
−
1
−
1
2
1
−
1
d
=
(
+
)
=
(
+
+
)
d
=
(
+
)
and
>0
so
<
2
2
d
2
1
σ
2
2
σ
2
0
σ
2
1
σ
2
2
σ
2
0
σ
2
1
σ
1
n
σ
2
2
(
µ
+
x
)
⋅
d
α
In
2
,
<
. It is clearly that because of the addition of new
1
0
2
0
2
1
σ
sample, the proportion of original prior and old sample declines. According to
equation (6.9), with the continuous increase of new sample (here we assume the
sample size keeps invariant), we have:
n
σ
1
n
σ
n
2
α
=
2
m
(
µ
+
x
2
x
x
⋅
d
+
+...+
)
m
1
0
2
m
σ
2
0
2
1
2
σ
m
m
n
1
+
Ã
=
2
m
(
µ
x
)
⋅
d
=
(
k
= 1,2, ... ,
m
)
(6.10)
2
0
0
k
σ
2
σ
k
1
k
From equation (6.10), if the variance of new samples are same, they equal to
a sample with the size of
. The above process weighted all the sample means
with their precisions. The higher the precision, the bigger the weight. If the prior
distribution is estimated precisely, we can use less sample data and only need a
little computation. This is especially useful in the situation where sample is hard
to be collected. It is also the point that Bayesian approach outcomes other
m×n




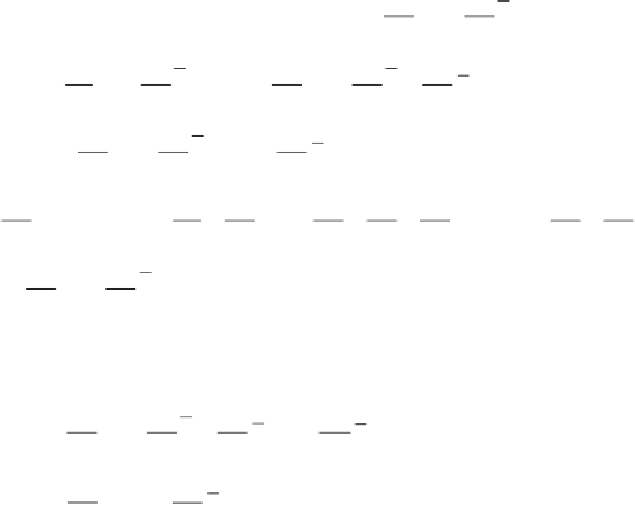