Biomedical Engineering Reference
In-Depth Information
Fig. 7.2
Illustration of the difficulties due to averaging signals across trials. (
a
) six trials of
simulated EEG, comprising two events that are subject to variablity in latency, amplitude and
frequency. (
b
), (
c
)and(
d
): Averaged signal with various time alignments. (
b
) no time alignment,
(
c
) time alignment on the
left
event, (
d
) time alignment on the
right
event
Outline
This chapter deals with the analysis of multitrial MEG or EEG dataset, and
presents two classes of approaches: data-driven, and model-driven. In Sect.
7.2
we present a data-driven approach for dimensionality reduction which allows to
reorder the trials, and subsequently simplifies their analysis. In Sect.
7.3
we present
a multitrial version of Matching-Pursuit, which models the signals of interest as
linear combinations of atoms from a predefined dictionary.
7.2
Data-Driven Approaches: Non-linear Dimensionality
Reduction
Considering a dataset described by the additive model (
7.2
), statistical methods such
as Principal Components Analysis (PCA) can be used to explore the structure of the
trial-dependent activity
x
k
(
is not too large.
We consider multitrial datasets that lie on a noisy 1-D manifold. This often
occurs in multitrial ERP recordings, in which similar neural activations occur across
t
)
, if the additive noise
n
k
(
t
)
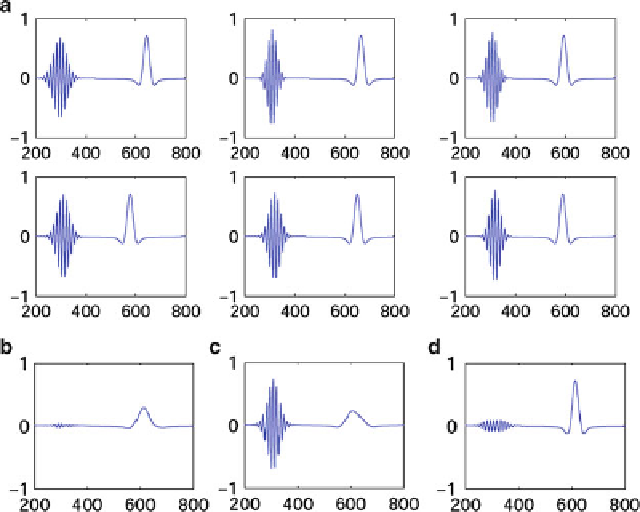
Search WWH ::

Custom Search