Civil Engineering Reference
In-Depth Information
Fig. 6
Example of a Pareto
set
of a residential building. Diakaki et al. (
2008
) developed a MOP model to find
alternative measures for improving energy efficiency in buildings. Hamdy et al.
(
2011
) proposed a MOP approach based on genetic algorithm (GA) to tackle the
problem of designing low-emission cost-effective dwellings. The proposed
approach is used to minimize the carbon dioxide emissions and the investment cost
for a two-story house and its HVAC system. Magnier et al. (
2010
) used a simu-
lation-based artificial neural network (ANN) to characterize building behavior, and
then combined ANN with multi-objective GA for optimization of thermal comfort
and energy consumption in a residential house.
Multi-objective Programming (MOP) Approaches using GA
The use of GA to deal with MOP models has gained an increasing relevance due to
their ability to work with a population of individuals (solutions) that expectedly
converges to the true non-dominated front (Deb
2001
). GA are particularly suitable
for tackling hard combinatorial and/or non-linear models, as they are less suscep-
tible to the shape or continuity of the non-dominated front than the classical
(mathematical programming) optimization methods. The rationale is that GA deals
with a population of solutions, and the aim is generally the characterization of a
non-dominated front. In this setting, GA-incorporating techniques to preserve the
diversity of solutions (for a comprehensive depiction of that front, thus unveiling
the trade-offs in different regions of the search space) possess advantages compared
with the use of ''scalarizing'' functions, in which a surrogate scalar function
aggregating the multiple objectives is optimized, as in traditional mathematical
programming approaches. However, it must be noticed that, in real-world prob-
lems, this is, in general, ''just'' a potential non-dominated front (also known as
Pareto front), classified as such because no other solutions dominating it could be
found but no theoretical tools exist, which guarantees their true Pareto optimality.
GAs have been extensively used as search and optimization tools in several
real-world problems, such as building energy efficiency problems, due to their
flexibility and good performance in exploring the search space. Regarding building
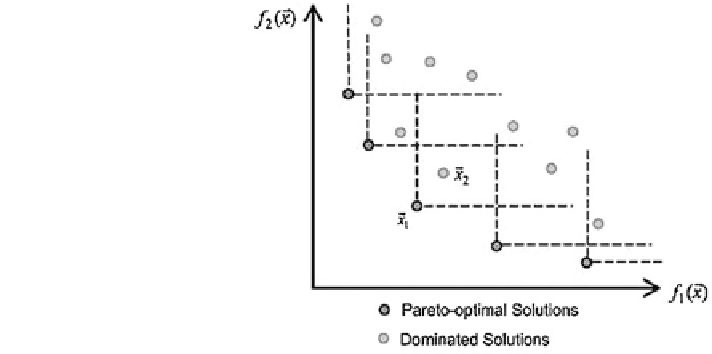