Biology Reference
In-Depth Information
4.4. Probabilistic Methods
Probabilistic methods (statistical phylogenetics) explicitly define a prob-
abilistic model of phylogenesis. At a minimum, each character is attrib-
uted a matrix of probabilities for the transformation from one state
to another, at any point on the tree. More complex models include
variations of mutation rates between sites, explicitly modeled insertion/
deletion events,
7,8
and even horizontal gene transfer.
It is then possible to estimate the statistical likelihood of an evolu-
tionary scenario of transformation of the characters, from root to leaves,
for each conceivable tree (topology and branch lengths).
Two main classes of algorithms are used to evaluate the likelihood of
a given tree. The first one consists of maximum likelihood (ML) meth-
ods, which are generally combined with more or less straightforward hill-
climbing algorithms. The second class relies on a Bayesian framework
and is frequently coupled with Markov chain Monte Carlo (MCMC)
algorithms; these Bayesian inferences are also probabilistic in nature, but
they proceed by refining a model according to the available data. On
occasion, Metropolis-coupled Markov chain Monte Carlo (MC)
3
meth-
ods are also used.
q
Using a probabilistic model has many advantages. Heterogeneous rates
of evolution between branches and between sites as well as homoplasy are
dealt with explicitly because they are inherent to the models. Statistical eval-
uation of the results is simple, because the probability of the best calculated
tree actually having arisen under the chosen model of evolution can be given
directly. Furthermore, probabilistic methods can be used to verify whole
new classes of hypotheses, which are beyond the scope of classic methods.
Which model of evolution best fits the data? Does the data fit a model of
evolution which allows for recombination? If so, which parts of the
sequence underwent recombination? All of these questions become possible
because likelihoods under various models can be compared and models can
be optimized for the dataset being studied.
On the downside, model-based methods generally are very compu-
tationally intensive and — as for CMP methods — no guarantee can be
q
For a detailed review of these methods, their advantages, and their drawbacks,
see Holder and Lewis.
9
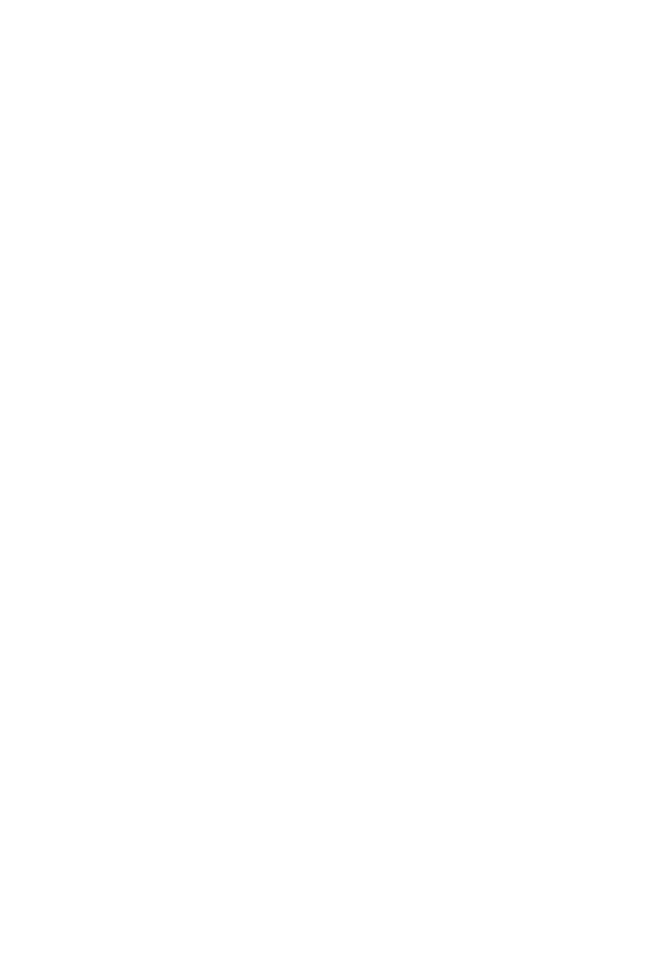

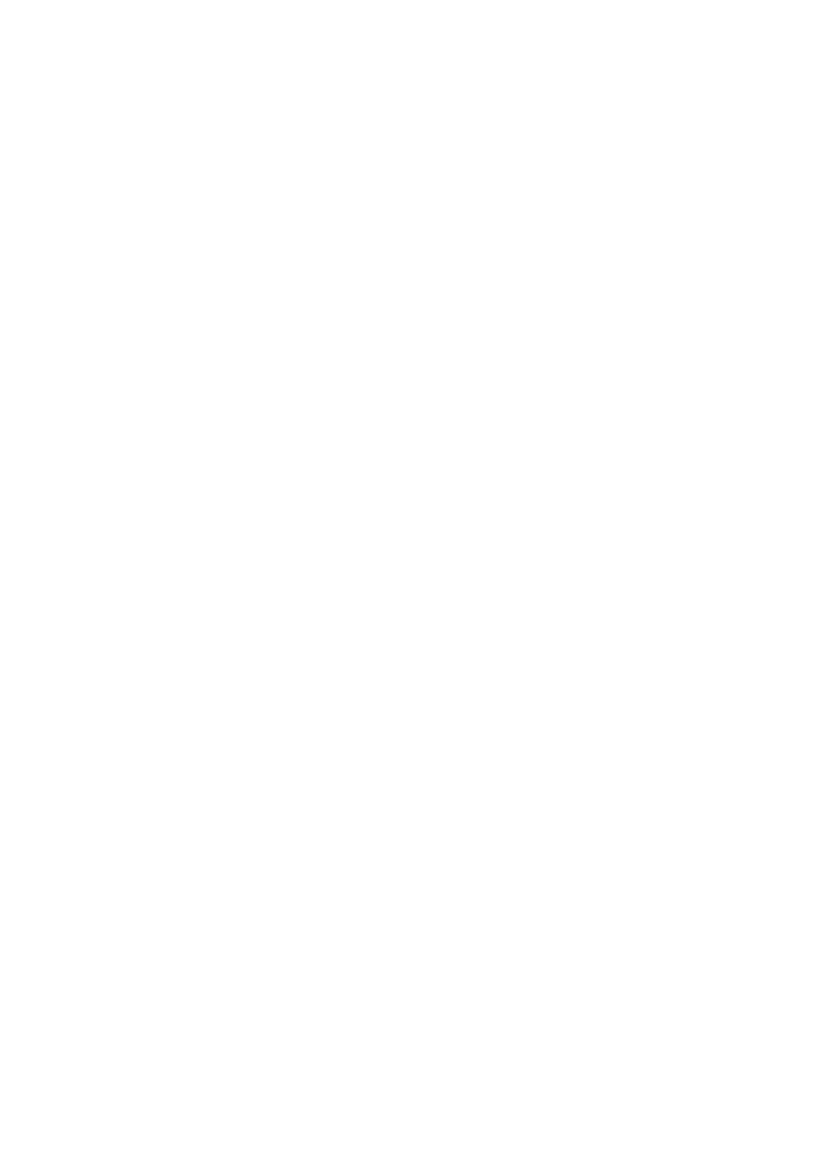