Information Technology Reference
In-Depth Information
Fig. 3.8 Correction of the
posterior probability in
classification for different
dimensions and residual
dependencies
20
D=2
D=3
D=4
D=5
18
16
14
12
10
8
6
4
2
0
1
1.1
1.2
1.3
1.4
1.5
1.6
1.7
1.8
1.9
2
I
RD
estimation of the source pdf's performed well for three and four dimensions. The
higher the values of residual dependencies, the greater the improvement in clas-
sification by the correction of the posterior probability. In addition, the improve-
ment was better in the higher four-dimensional space since one- and multi-
dimensional estimations were more differentiated when the number of dimensions
was increased. For two dimensions, the behaviour of the correction was not
consistent for different ranges of residual dependencies, performing worse for
higher values [1.52-1.8] of I
RD
than for lower values [1.29-1.42] of I
RD
. However,
the correction always improves the classification results in all the explored ranges
of residual dependencies. The varying results in the two-dimensional space were
due to similar achievements by the one-dimensional and multi-dimensional esti-
mators for lower dimensions. In the case of five and higher dimensions, a higher
number of observation vectors was required for a precise non-parametric esti-
mation of the data distribution. The results for D
¼
5 were obtained by increasing
the number of observation vectors per class from 200 to 1000. In this case, the
tendency of the curve was correct, but the improvement in classification was lower
than for D
¼
3 and D
¼
4. A much higher number of observation vectors was
required to obtain better results than those used in lower dimensions.
3.4.5 Semi-supervised Learning
The proposed procedure includes learning with both labelled and unlabelled data,
which is called semi-supervised learning. Statistical intuition advises that it is
reasonable to expect an average improvement in classification performance for any
increase in the number of samples (labelled or unlabelled). There is applied work
on semi-supervised learning that optimistically suggests the use of unlabelled data
whenever available [
27
-
30
]. However, the importance of using correct modelling



















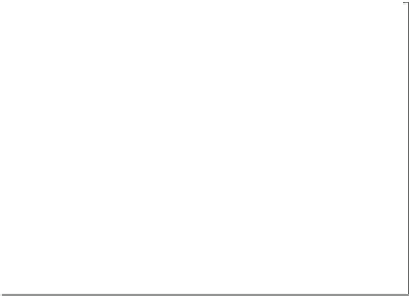
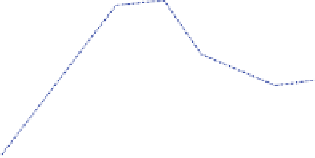



























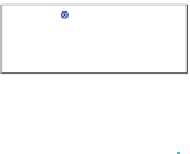
















































Search WWH ::

Custom Search