Information Technology Reference
In-Depth Information
Fig. 3.2 Second simulation
experiment—BSS in ICA
datasets
40
35
30
25
20
Mixca
FastIca
JADE
InfoMax
Extended InfoMax
Radical
Npica
Kernel-ICA
15
10
5
0
500
1000
1500
2000
2500
3000
3500
4000
4500
5000
N
random variables (at most one Gaussian) by approximating their independence
with a distance measure (contrast) obtained with higher order statistics.
A total of 300 Monte Carlo simulations of the second experiment were per-
formed for each of the values of N
¼
500
;
1000, 3000, 5000. The mean results of
the second experiment are shown in Fig.
3.2
.
The mean results for the second experiment (Fig.
3.2
) show that non-parametric
ICA algorithms outperform more conventional ICA methods. The average per-
formance difference between the first three non-parametric algorithms is below
1.8 dB. The Mixca algorithm conducts a consistent separation improvement for
different observation vector sizes, and it is able to learn the source densities for
even a small number of observation vectors (500). JADE and FastIca deliver a
similar performance loss with non-parametric Mixca of over 6.5 dB for all the
different observation vector sizes. Extended InfoMax and InfoMax have worse
separation with smaller observation vector sizes (N
¼
500
;
1000 observation
vectors). They achieve a performance loss over 16.9 and 16.4 dB, with respect to
non-parametric Mixca. For larger observation vector sizes (N
¼
3000
;
5000
observation vectors), the performance loss with non-parametric Mixca is over 7
and 11.4 dB. Higher order statistics methods exploit the non Gaussianity of the
sources in order to obtain the solution. However, they do not consider the time
structure of the signals, i.e., the sources are considered random variables, and not
time series.
Figure
3.3
shows detailed results of the separation for each of the sources in
Table
3.4
. In general, non-parametric ICA algorithms consistently outperform the
standard ICA algorithms for all the sources with higher gain for skewed sources
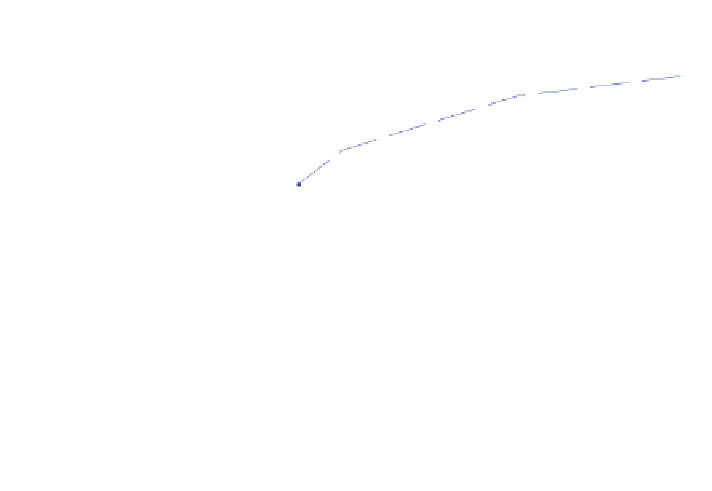


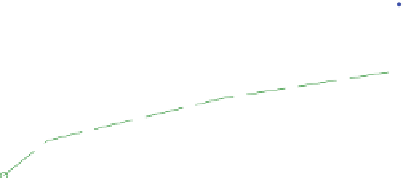



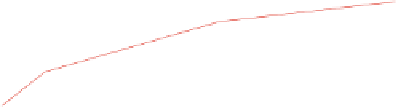




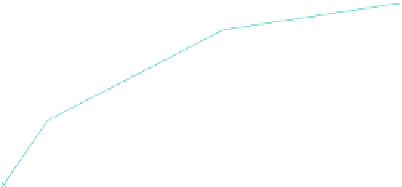


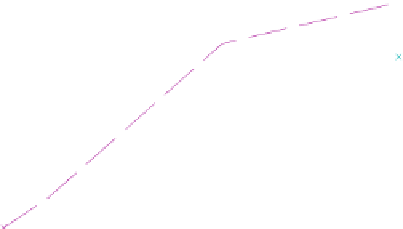



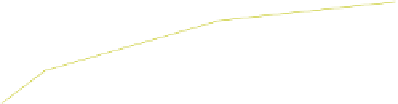



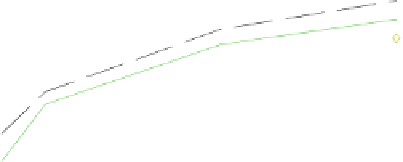



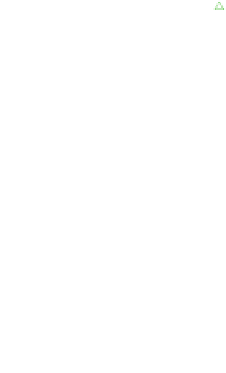


































































































































Search WWH ::

Custom Search